Artificial intelligence is often considered the solution to many of society’s pressing problems. It can sort through data at lightning speed, make accurate predictions, triage more effectively than humans – the list goes on. But it remains a flawed, evolving technology, and recently AI hallucinations have taken center stage in discussions about it.
It makes odd suggestions, like using glue to prevent cheese from sliding off pizza and it invents entire court cases when asked to perform legal research. Yet despite these hallucinations, AI is still a heavily relied-upon tool: today, companies, governments and entire industries use it as the backbone of their operations.
But how can a tool that so often creates erroneous results be used for industrial purposes that require the utmost precision? The answer is a bit complex.
What are AI hallucinations?
AI hallucinations are inaccurate or misleading generations that may be produced for a number of reasons, including processing mishaps and poor training data.
Take Google’s AI Overviews, for example. Rolled out recently, they began hallucinating almost immediately, suggesting users eat rocks to stave off mineral deficiency or jump off the Golden Gate Bridge. Almost as quickly as the overviews appeared, Google walked many of them back.
“Large Language Models (LLMs) are trained on a large body of public texts, mostly from the internet. Their learning environment will naturally include a lot of generic information, some of which is junk,” explained Eric Vernet, a natural language processing expert and AI specialist at Dassault Systèmes.
“Having all of that knowledge – both useful and misleading – can lead to completely false answers in some cases,” he added.
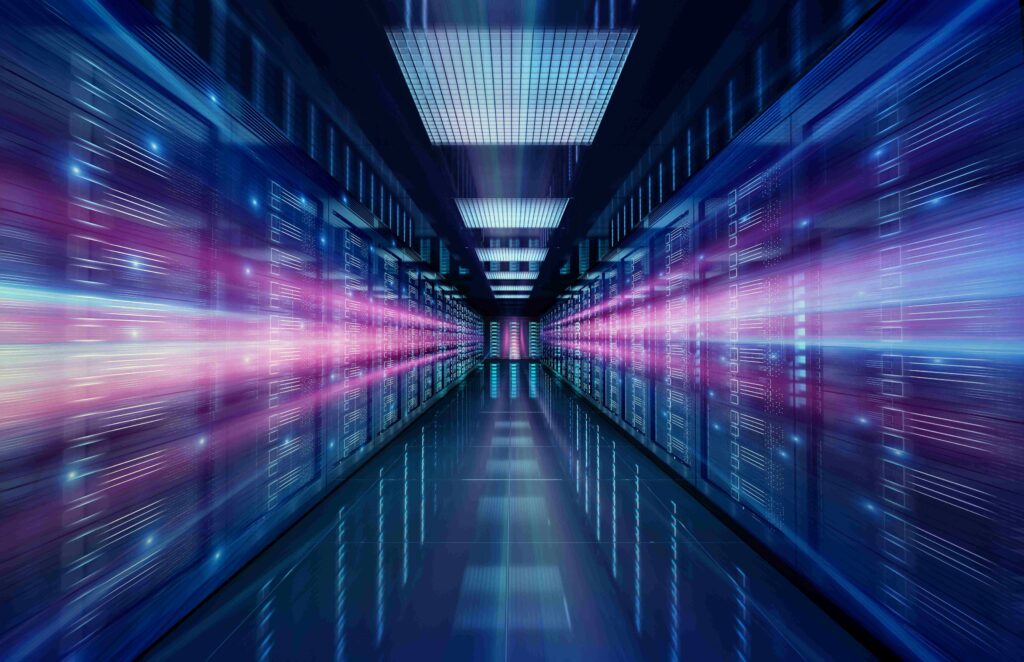
Like most technology, AI has weak spots, and generative AI tools are particularly vulnerable. AI doesn’t think per se; it sorts. It looks at large amounts of data, identifies patterns and infers truths from them. It doesn’t have a mind of its own to consider the weight of the ideas it spits out. And when it intakes massive amounts of information – some of it junk – it doesn’t know how to differentiate between right and wrong.
Explaining the Google overview snafu, the company’s VP of Search, Liz Reid, said the product is trained to “show information that is backed up by top web results,” though the popularity of a given web page doesn’t mean what’s written on it is correct.
One of AI’s greatest pitfalls is that it can only be as smart as the data it intakes, and if that information isn’t high quality, then the output won’t be either.
Helpful despite hallucinations: AI beyond chatbots
Evidently, generative AI is flawed. But there are plenty of other types of artificial intelligence out there, and those are the ones that businesses rely on to conduct their everyday operations.
A car manufacturer, for example, might use discriminative AI to classify components and then couple it with predictive AI, which can identify patterns and make predictions to be leveraged for decision-making. Those predictions can apply to anything from material costs to machine maintenance – at every level of the business’ operations, there’s a use case for AI.
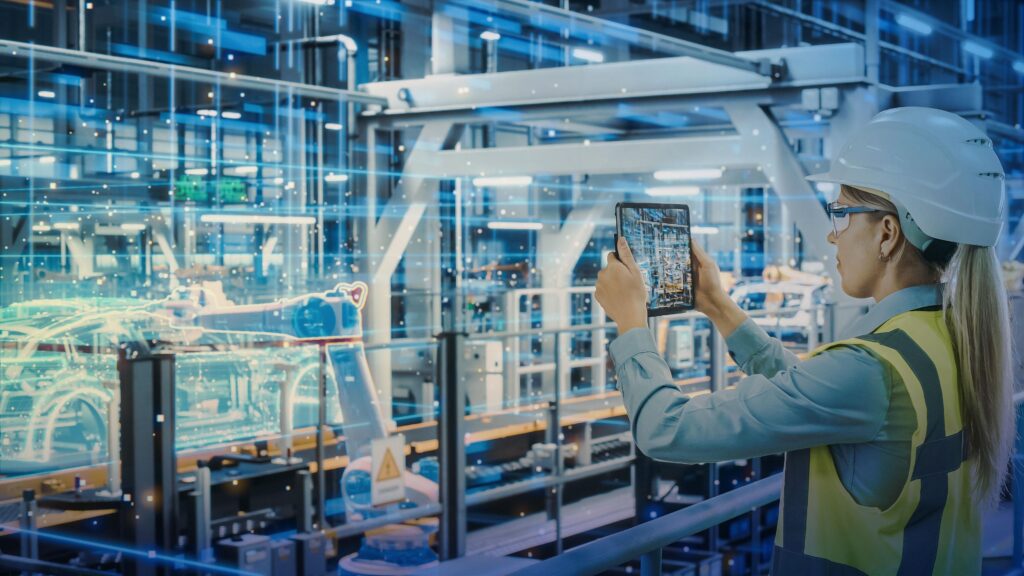
These types of discretionary tools are more accurate, more useful and more successful than publicly available programs, and it’s all because of how they’re trained.
While public-facing programs are trained on LLMs, the AI used for industrial purposes isn’t. It learns in a silo that contains only the information the tool needs to have in order to be useful, no more and no less. Because it’s only exposed to relevant, hyper-specific information, it’s less likely to hallucinate.
“Back-end AI that’s trained on a company’s own private data and taxonomy is a lot more accurate” than its generative counterparts, said Vernet. “Some companies have their own specific vocabulary and processes and the like, so if an AI is trained specifically on those sets of information, it will be ‘smarter.’”
This is especially pertinent for companies with significant proprietary information or which use highly specific internal language. Deploying tools trained on public data wouldn’t be able to achieve what it needs to. Only a unique one that’s familiar with their terminology, acronyms, processes and more will accomplish what it needs to.
Can AI hallucinations be fixed?
Like any technology, AI requires what NLP expert Kelly Stone calls the “human in the loop” validation stage. Including humans in the process both to check and refine AI output will ensure that this type of technology continues to learn so that, down the line, it’ll produce even more refined and accurate answers.
AI companies are continuously working to improve their products, and there are a few key ways that these improvements are typically made. Having an abundance of data is a formidable start, but structuring that data in a way that it’s easily accessible and usable is the real challenge. And considering that most companies’ data constantly grows – and sometimes does so exponentially – means a robust structure is incredibly important.
Maintaining databases in a singular location, like the 3DEXPERIENCE platform, enables companies to keep their information in a consistent setting and state. From there can this data be effectively leveraged for any type of function, whether it’s predicting cost fluctuations or performing NLP to contextualize market trends. Moving away from siloed information, the managed platform gives users access to advanced analytics in supply chain that have the potential to optimize processes, uncover new opportunities and transform business operations for the better.
Stone, of Dassault Systèmes’ information intelligence brand NETVIBES, advocates further development and usage of programs like these. Refining AI will reduce hallucinations, resulting in more trustworthy tools for users of all kinds. Combine that with continued expansion of back-end programs and the AI that fuels them, and you’ve got a perfect storm of intelligence.
“I do kind of believe there’s a future where AI is incredibly reliable,” Stone said. Though she admits we’re not there yet, she thinks the prospect of all kinds of AI – generative, discriminative and predictive alike – being closer to 100% accurate instead of 80% or so isn’t unimaginable.
Absolute excellence is impossible: as humans, we can’t even achieve that, so attempting to create a machine that would be able to be is a bit far-fetched. But overcoming hurdles like generative AI hallucinations will open up a world of possibilities that will enable us to realize the full promise of this type of emerging technology.
Perfecting problem-solving for the future of AI
Just like every other technology and innovation, AI has a bit of a last-mile problem.
Bus lines end at a certain point, yet passenger journeys continue on. Prohibitive roadways and malfunctioning GPS devices prevent deliveries from landing on doorsteps, yet we continue to order goods online. Artificial intelligence is no different. Its generative capabilities are those last miles, the neighborhoods not yet served by the subway system or the remote areas reached only by drone.
We didn’t get rid of public transportation systems when it turned out they didn’t serve every single city block, and we haven’t scrapped the idea of online ordering just because some addresses are hard to get to. We’re still working to make these opportunities available to all, and AI’s rocky path toward near-flawlessness reflects that same process.
While generative AI might continue to hallucinate, its back-end counterparts work tirelessly to uphold entire industries. Continuous refinement, training, and exposure to high-quality, structured data will be necessary to ensure artificial excellence. The last stretch to perfection – the nuance, the inexplicable human touch, the grappling with unforeseen scenarios – often proves elusive. But elusive isn’t impossible. While the phenomenon of AI hallucinations presents a unique set of challenges, it also highlights the critical importance of human collaboration in the development and deployment of AI.
As companies and technologists continue to develop strategies for improving AI’s performance, the optimism surrounding its capacity to transform industries only grows stronger. The journey toward refining AI is fraught with complexities, but the quest to bridge the last-mile gap is a testament to the collective ambition to shape a future where AI solutions are not just nearly perfect but are trusted, reliable, and an integral part of solving some of the world’s most pressing challenges.