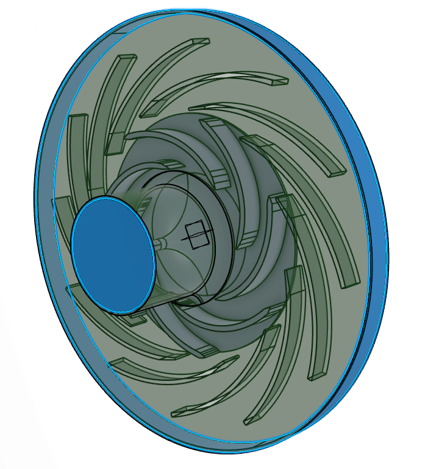
Design & SimulationOctober 17, 2024
Machine Learning Accelerates Centrifugal Pump Design
In this blog post, we show how machine learning can be used in the design process of a centrifugal pump to optimize diffuser geometry. The AI model is trained on data from best-in-class simulation tools which produce reliable data that closely agrees with experimental validation results. Using a trained AI model based on simulation data, engineers can explore the impact of changes to diffuser parameters with instant feedback.
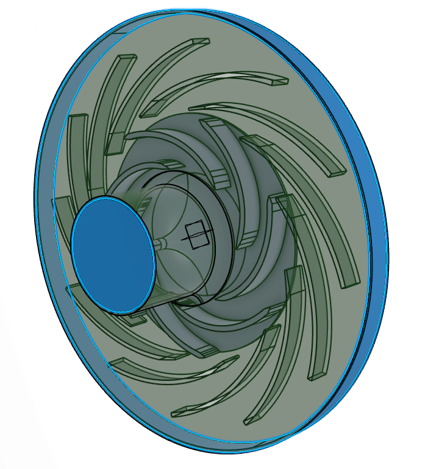
Stay up to date
Receive monthly updates on content you won’t want to miss