In 1950, British mathematician and computer scientist Alan Turing posed the question: “Can machines think?” While computing was in its infancy at the time, it is integral to our lives today. Despite this technological revolution, Turing’s question still remains: How can we make machines intelligent enough to think and communicate effectively with us?
Many of us are familiar with chatbots or virtual assistants. These applications pop up when visiting a website, offering help or answering questions. According to a survey by chatbot service provider Tidio, 52% of consumers use chatbots to answer simple queries, and 12% use them to avoid the stress of speaking with a human agent.
However, chatbots and virtual assistants can also cause frustration – even aggravation. In fact, a report from Statista shows that 47% of internet users in the US feel chatbots provide too many unhelpful responses.
Clearly, the natural language processing (NLP) models and artificial intelligence (AI) powering these virtual assistants is not yet sophisticated enough to fully understand the nuances of human language. Why? François-Régis Chaumartin, founder and vice president of data science at NLP software company Proxem, now a part of Dassault Systèmes, believes it’s because human language is intrinsically complex.
AI is not true intelligence. Chatbots are very close to passing the Turing test. But so far, they are simply the best stochastic parrots that humanity has ever created.
François-Régis Chaumartin
founder and VP of Proxem
“As human beings, we forget how difficult it is to learn and understand language because, from childhood, we have parents and teachers to continually teach us,” he explained. “We can try to mimic language understanding in chatbots with programming, but we can only do this in very restricted scenarios of the world. The machine is programmed to wait for given keywords, but it has absolutely no general understanding or common sense.”
HOW DO ROBOTS LEARN NLP?
AI models, such as those used by robots to help them talk to humans, are taught in two different ways: rules-based and data-based. In rules-based scenarios, the AI is taught a set of rules, which it then applies to the data it receives.
“This works very well in specific data sets where there are very few exceptions to the rules, but this is rare in human language,” said Dimitar Kazakov, a senior lecturer in the department of computer science at the University of York, UK.
Chaumartin agreed: “There are limitations with rules-based systems because there are exceptions in human language, and then exceptions to those exceptions.”
READ: Natural language processing helps organizations take a more human-centric view
The data-based approach bypasses these issues by training AI on huge amounts of data – human language from the real world, in the form of text. “This helps the machine to understand the grammatical rules of a given language, without needing explicit instructions,” Chaumartin said.
Language is not just a conveyor of meaning between individuals. It gives you the means to understand the world around you.
Dimitar Kazakov
lecturer at University of York, UK
Using both these methodologies helps a computer demonstrate near-human intelligence on specific subject areas.
“The narrower the purpose of that chatbot and the topic of its conversation, the better it will be when conversing with humans,” Kazakov said. “It can be a simple case of a statistical model, so that when the AI detects a certain attribute associated with the meaning of a word, phrase or sentence, it can find close matches in the data it was trained on, enabling it to respond correctly to a query.”
A QUESTION OF ETHICS IN NLP
A data-based approach to natural language processing enables researchers to train AI using increasingly large language models. Models can be taken, for example, from the internet and sites such as Reddit, Twitter and Wikipedia, where anyone can contribute. In theory, these could better prepare AI to effectively communicate with humans thanks to the diverse and nuanced language used.
However, researchers at the University of Washington in Seattle, and from the tech industry, have pointed out that vast models like these could actually have the opposite effect: Large datasets based on texts from the internet over-represent the loudest voices – including trolls – and can encode biases that are damaging to marginalized populations.
The authors of this paper, titled On the Dangers of Stochastic Parrots: Can Language Models Be Too Big?, recommend that NLP researchers carefully consider the risks of their work.
“We call on the field to recognize that applications that aim to believably mimic humans bring risk of extreme harms,” they wrote, noting that ethical AI development requires considering and modelling downstream effects to limit harm to different social groups.
Other research, for example that of Manzini et al. in their 2019 paper titled Black is to Criminal as Caucasian is to Police: Detecting and Removing Multiclass Bias in Word Embeddings, suggests that those working in the field can go one step further, proactively removing problematic language from datasets to prevent the spread of biases and stereotypes.
Robots come with other risks as well. For example, one study found that an overreliance on GPS systems may inadvertently put people at higher risk for dementia. Another, titled Easily Accessible but Easily Forgettable: How Ease of Access to Information Online Affects Cognitive Miserliness, found that instant access to information – a potential outcome of increased AI use – is affecting our ability to retain information. What’s worse, these unintended consequences happen in situations where the AI is working exactly as it should.
SMALL-TALK SPECIALISTS
Despite such potential complications of AI in conversation with humans, Kazakov believes that current NLP models are capable of conducting casual conversation or small talk.
“There are programs that can look for patterns and keywords in the text and use simple syntactic transformations to take what someone says and bounce it back with ‘is that so?’ or some other low-level conversational retort,” he said.
This ultimately answers Turing’s 1950 question. His namesake test – originally called the imitation game – assesses intelligence by measuring whether a human can distinguish conversation with the machine from conversation another human. A recent example: in June 2022, Google engineer Blake Lemoine stirred up controversy when he went public with his views that Google’s Language Model for Dialogue Applications (LaMDA) had achieved consciousness and should be treated as a Google employee. In a blog post, he wrote: “LaMDA has been incredibly consistent in its communications about what it wants and what it believes its rights are as a person.”
However, believing that AI has consciousness does not necessarily mean the computer has reached human-level intelligence.
“AI is not true intelligence,” Chaumartin said. “In recent months, we have seen great breakthroughs using deep learning, by training AI on hundreds of billions of web pages to understand human languages. These chatbots are very close to passing the Turing test. But so far, they are simply the best stochastic parrots that humanity has ever created.”
CHECK OUT: How NLP improves information intelligence
Chaumartin’s stochastic parrot – a system of stringing together words and phrases based on rules and training – emphasizes that AI only repeats things that have already been written. “It is really impressive, and it is getting better at mimicking human intelligence each year, but ultimately that is all it is – mimicry,” he said.
In other words, we can teach robots to talk; the hard part is getting them to understand. For Chaumartin, this difference stems from something innate to humans.
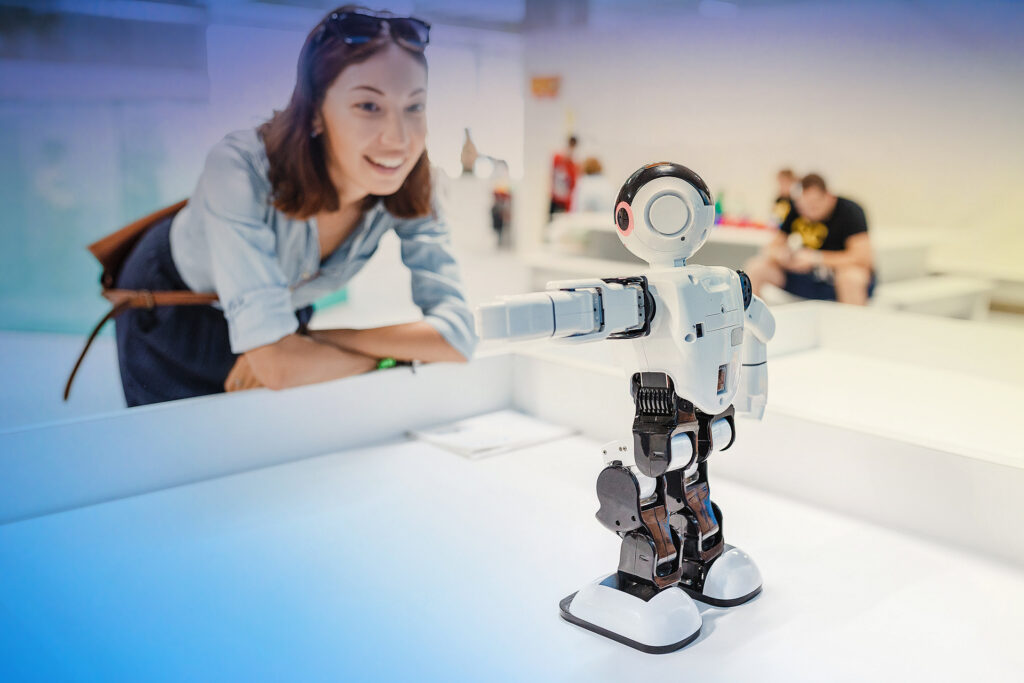
“This is the magic of biological evolution,” he said. “Humans are the result of hundreds of millions of years of evolution and 100,000 years of language. It has made the human brain able to learn with very little information, and that is a key difference for machines today – they need huge quantities of information to learn something, though no one knows exactly why.”
DEVELOPING A SURVIVAL INSTINCT
“It has been suggested that perhaps it is the fight-or-flight instinct that makes humans and animals more intelligent than machines,” Chaumartin said. “We must survive in dangerous environments, and this helps us to hone our creativity.”
Researchers are exploring ways to help machines become more intelligent by accessing the motivation created by fear and survival in a field called grounded NLP. “This experimental research aims to help machines experience senses, stress and danger, and to learn faster and more efficiently,” Chaumartin said.
While current AI models can understand words, they cannot necessarily understand the overall context and meaning conveyed by language, let alone tone of voice. According to Kazakov, this too is what separates humans and machines.
“Language is not just a conveyor of meaning between individuals. It gives you the means to understand the world around you,” he said.
PAINTING A PICTURE WITH NLP
Some engineers are working to help AI reach this greater level of understanding by training models on multimodal data: text and imagery linked to the same underlying concept. DALL-E is a fun example of this. The machine learning model, developed by AI research lab OpenAI, generates digital images from natural language descriptions.
“The magic of DALL-E, and all these recent language and image models, is to do the learning simultaneously on text and images, to have the same shared representation,” Chaumartin said. “When you close your eyes and you think about an apple, you think of the word and the image at the same time. The same thing is now possible for AI.”
AI models like this could create a whole range of new opportunities in design, media and filmmaking. “If you start inputting text tags that you haven’t seen before, you’ll start seeing images that you haven’t seen before, which is incredibly impressive,” Kazakov said.
WHAT’S NEXT?
The lack of true intelligence means that AI will, for the time being, remain under human supervision. A good example: automating some of the many labor-intensive and administrative tasks previously carried out by humans in fields like data analysis and answering simple queries.
Robots have already replaced many human roles, most obviously in manufacturing, and more will likely follow. For example, Japanese automation company AIST has created a robot called PARO that aims to provide the benefits of animal therapy to hospital patients by reducing stress and improving socialization – without using actual animals. A UK-based team of researchers, meanwhile, has designed an AI model that can predict heart disease with greater accuracy than doctors. Another example: AI being used to identify knowledge gaps in school children and personalize curricula to suit their needs.
However, our ability to identify and remove ethical biases in the datasets used to train AI models will become increasingly important as we look to robots to take on more direct interactions with humans.
“While there is not yet a universal way to check for the biases encoded in data sets, we have made significant progress in the past decade,” Chaumartin said. “Ten years ago, no one was paying much attention to the ethical considerations around AI, but that is clearly changing. The European Union is even working on implementing law to improve AI transparency.”
For AI’s evolution to continue, NLP researchers must understand the risks associated with their work and train their models to navigate the nuances of complex human conversation, ensuring successful and safe interactions with people.
“As NLP advances and robots get more intelligent, there is enormous potential for them to positively impact people’s lives,” Chaumartin said. “Their ability to help us is limited only by our ability to teach them.”