In this new post of Artificial Intelligence (AI) for Resource Estimation, we examine early Machine Learning applications and pilot studies and how they might affect the mining industry in the near term.
Studies show that Machine Learning (ML) techniques, when integrated with traditional geostatistical techniques, save time, reduce costs, and improve prediction accuracy.
Over the past 15 years new mineral deposits have become increasingly difficult, expensive, and risky to discover. In response, engineers and data scientists are developing new geological data-collection tools that generate enormous, continuous volumes of data that geologists and mining engineers can use to improve log drill cores, predict lithofacies from geophysical logs, predict mineralization, and optimize drilling and exploitation.
The challenge is integrating all this data with traditional geostatistical resource estimating techniques.
Hybrid Approach
Geologists currently use two common methods for geology interpretation. One requires interpretation of geology in sections by digitization. From these sections, a 3D model is constructed using triangulation. Another is implicit modelling (based on radial basis function). It has become widely used to construct orebody models by interpolating drillhole data directly.
For resource modeling, most geostatistical algorithms have already been automated in modern mineral resource software packages. The industry commonly uses simple univariate geostatistical models such as Inverse Distance Weighting and Kriging for resource prediction.
Data scientists are developing ML to integrate more data from multiple sources
into traditional geostatistical models to find, evaluate, predict, and map subsurface rocks. ML models have the potential to dramatically cut drilling costs, ensure early availability of geology data, reduce human error, increase prediction accuracy, slash overall costs, and shrink exploration lead time.
More Data, Better Models
Machine Learning is beginning to be deployed in some limited mining applications.
Continue reading the full exclusive article on the GEOVIA User Community.
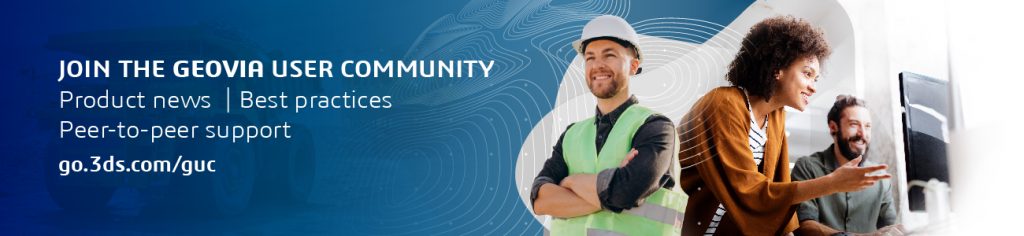
The GEOVIA User community: a community by and for GEOVIA users
Discover the community of reference for GEOVIA peer-to-peer support, materials and expert insights!
- Get started and make the most of your GEOVIA software
- Become an expert in your domain of interest
- Share your knowledge and experience
- Get help from experienced users
- Discover the Virtual Mine Experience
New member? Create an account, it’s free!
Learn more about this community HERE.