In this new post from the “AI for resource estimation” series, we drill deeper into how data scientists and geologist make machines smart enough to see more of what’s underground with less drilling.
Machine Learning (ML) models help geologists visualize the features of rocks that would otherwise remain invisible deep underground.
The problem with drilling is that, while it provides relatively reliable ore samples for analysis and validation, it’s expensive, slow, and cumbersome. Core drilling to obtain physical samples for lab analysis, data consolidation and reconciliation, validation, modeling, and publishing can take up to 17 weeks.
Machine Learning that correlates massive data can do it in one. And predict ore qualities with very high confidence, cheaper, and with less drilling. So, how do you make a machine smart?
Teachable Machines Instead of Core Drilling
Artificial Intelligence (AI) is a catchall term that includes Machine Learning, neural networks, deep learning, computer vision and natural language processing. AI generally refers to the broad idea that machines can execute tasks “intelligently.”
Machine Learning refers to the concept that machines can learn and adapt through experience. A neural network is a computing system modeled after the human brain. Deep learning uses huge neural networks with many layers of processing networks. Computer vision uses pattern recognition and deep learning to recognize what’s in a picture or video. Natural Language Processing allows computers to analyze, understand and generate human language and speech.
Data scientists often assemble and combine different AI approaches in various ways to quickly interpret oceans of data no single person or team can manage alone. It’s about pattern recognition across disparate databases from wide-ranging sources: government geological records, site equipment sensors, borehole samples, satellite imagery, magnetic field readings, topographical maps, and field reports.
Machine Learning models for resource estimation interpret correlations among geoscience data to create geological and resource models that rely less on expensive, time-consuming core drilling operations. Faster and less expensive non-core drilling…
Continue reading the full exclusive article on the GEOVIA User Community.
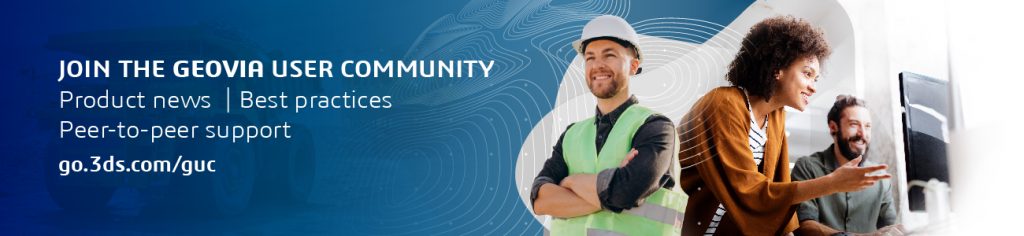
The GEOVIA User community: a community by and for GEOVIA users
Discover the community of reference for GEOVIA peer-to-peer support, materials and expert insights!
- Get started and make the most of your GEOVIA software
- Become an expert in your domain of interest
- Share your knowledge and experience
- Get help from experienced users
- Discover the Virtual Mine Experience
New member? Create an account, it’s free!
Learn more about this community HERE.