As a scientist with a theoretical physics and chemistry background, I sometimes get into conversations about the exciting (strange) world of quantum mechanics with (non-scientist) friends, talking to them about entanglement, Schrodinger’s cat, and wave-particle duality. Often, I get the reaction that “This is cool, but is there any real-world use for it?” The first responses that come to my head are along the lines of: “Yes, the atom exists because of quantum mechanics, otherwise, it would collapse; so our very existence is tied to it.” Or, “Semiconductors behave the way they do because of quantum mechanics, so electronics is based on quantum mechanics.” Recently, I can give another answer on top of my head too: we can design better batteries beginning with quantum mechanics and eventually ending up on a macro-scale, this is called multiscale battery modeling and what we do at our company, Dassault Systèmes. I work in the Contract Research team within the BIOVIA brand (one of the twelve brands in Dassault Systèmes). Below, I explain what makes our team unique, and why if you are a company working in the materials/life sciences space, you might want to consider us to design solutions for your challenges. So let’s talk about this a bit before going deeper into our journey from quantum mechanics to batteries.
What does the Contract Research team at BIOVIA do?
In short, we are a solution team. We connect the world of atoms and molecules to real-world outcomes: better products, cleaner energies, healthier communities, or more efficient industrial processes. Being part of BIOVIA and the greater Dassault Systèmes ecosystem, we have access to many proprietary materials/molecular modeling and data science commercial packages; in addition to that, we have the experts, the talents, and the diverse scientific/engineering background in our team, needed to translate software to a solution to a real-world hard challenge. Our team includes chemists, physicists, biologists, chemical engineers, materials, and computer scientists. As a team, we have graduated from some of the world’s best institutions and have vast postdoctoral and industrial experience. We have the curiosity and know-how of state-of-the-art science, coming from our academic background, combined with real-world practicality and problem-solving skills we have acquired through years of working in relevant industries or interacting with industrial clients. We have worked with and produced tangible valuable outcomes for some of the industry-leading companies in the energy, life sciences, chemicals, microelectronics, technology, consumer packaged goods, industrial equipment, aerospace, defense, and automobile manufacturing sectors. We have also received innovation awards from these companies for saving them cost and time and helping them accelerate product development, optimize processes, remediate failures and reach sustainability goals faster.
In a nutshell: what is a battery and what necessitates multiscale modeling
A battery is, fundamentally speaking, an electrical energy storage device. Let’s take Li-ion batteries as an example: when we charge such a battery, we inject energy into the system to put electrons and Li ions (Li+ cations) into a higher energy state. Then during the discharge, by putting the battery in a closed circuit, the Li+ cations and electrons reconfigure to their lower energy state again, generating energy (work) for the electrical circuit they are connected to. Thus, there are three main parts to a battery: the cathode (where Li+ migrates to during discharge), the anode (where Li+ migrates to during the charge), and the electrolyte (which allows for reversible migration of Li+ without letting electrons through). A simple schematic is included in Figure 1.
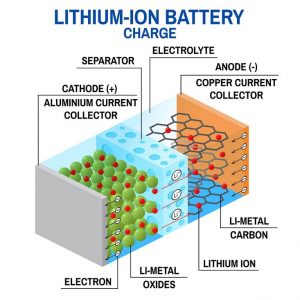
Figure 1 A: simple schematic of different components in a battery cell.
The reversible (rechargeable to be more exact) migration mechanism discussed above involves Li+ making and breaking bonds in the anode/cathode and diffusing through the electrolyte. These processes are quantum mechanical, as they involve the motion and interaction of particles on a sub-nanoscale. On the other hand, batteries are extremely complex devices; to just name a few of such complexities: a variety of materials with various (electrical and mechanical) properties and topologies form at the electrochemical interface between the anode/cathode and the electrolyte. Also, the electrolytes themselves are often a soup of different components. Therefore, to simulate such a battery, one cannot afford to use quantum chemical atomistic modeling to calculate the most important electrical or thermal properties of the system: it is unimaginably computationally taxing. That is what necessitates the multiscale simulations. To use higher precision (but more computationally expensive) approaches at smaller spatial and temporal scales, extract parameters of interest, then feed such parameters to less computationally expensive methods capable of performing at larger spatial and temporal scales. In the process, we need to use our physical and chemical intuition to “chop up” such complex systems into meaningful smaller pieces on which we can perform numerical experiments and extract useful information which can be plugged into other (larger scale) simulations. Doing such an exercise multiple times, at multiple scales, can bridge the gap between the nanoscale 3D quantum chemistry and the macroscale 3D-cell world.
What is the value that Contract Research can deliver?
The most important contribution from the Contract Research team (in addition to operating the BIOVIA’s software tools) is to provide the multidisciplinary expertise and experience to fill the (human and know-how) gap between real-world challenges and our proprietary software solutions at Dassault Systèmes. We break down the presented grand challenge into small but meaningful enough pieces. After simulating these smaller pieces, we can feed the results (derived parameters) into larger-scale (coarse-grained) simulations, and we eventually reconstruct the solution to the real-world challenge through what can be thought of as multi-scale problem-solving.
With the power of AI, computational power, and automation on an exponential rise, I constantly ask myself, will my job be soon replaced by AI or get fully automated? If I find the answer to this question positive, I would change my job right away! Fortunately, with our roles in Contract Research, we feel safe in this regard. The reason is that although we benefit from powerful software and AI as tools, our role (as scientists with chemical/physical/biological/engineering intuition, vast field knowledge, and experience) cannot be taken over by our tools (maybe it can be by the time we can go get a hot chocolate at a café on the terraformed Mars). To showcase the importance of our intuition and expert input on translating the power of BIOVIA’s solutions to better real-world products, in Figure 2, I have indicated a rough scheme of our interaction with the grand challenge from the beginning to the end, and have used green to reflect the parts of the process that are experts’-intuition-and-experience-heavy.
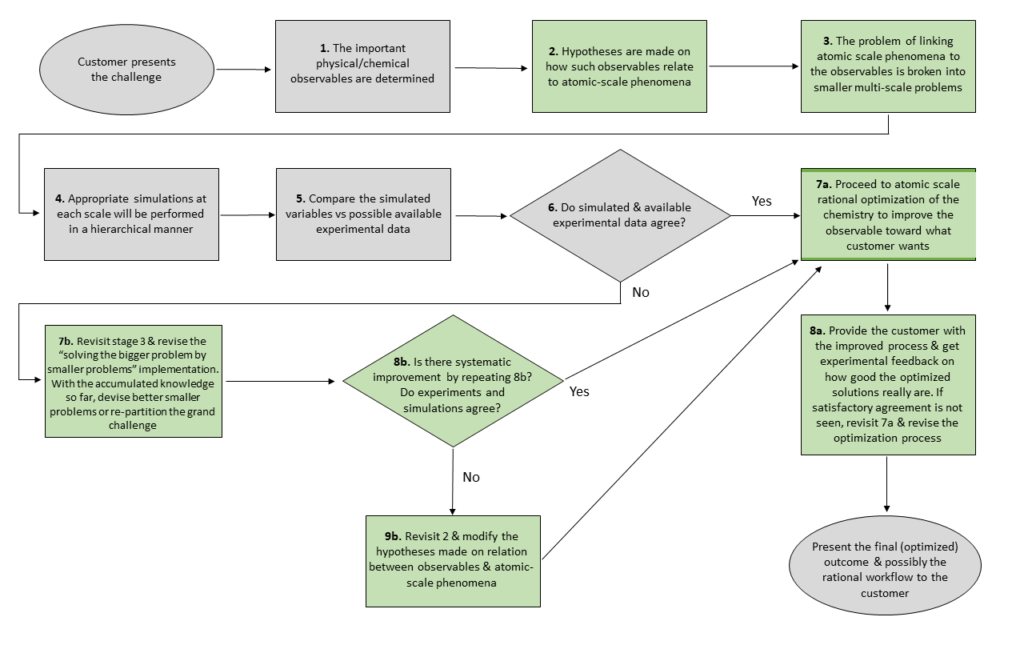
Figure 2: A schematic flowchart roughly depicting our interaction with the challenge we are hired to solve by our customers: from the real-world problem to smaller simulations, and to the outcome we deliver. I have used green to reflect the parts of the process which can greatly benefit from our experience and domain knowledge.
A few examples of the application of BIOVIA’s solutions in battery design
Below, to showcase the fun and power researchers can experience in applying BIOVIA’s solutions to battery design problems, I will discuss a few important topics in this area and review the tools provided by our software suites to address them. What has always impressed me using our software solutions at BIOVIA since joining the company, is how conveniently different modules and functionalities are integrated into a single user interface, enabling one to have a comprehensive virtual lab, 24/7, in an area as small as one’s laptop screen. The user can smoothly transition to different length and time scales, having the flexibility to implement multiple theories, approximations, and levels of chemical accuracy (often competing with calculation’s speed) to calculate the same parameter or chemical/physical observable. Such a powerful toolkit gives us researchers in Contract Research the ability to, not worry too much about coding in our tools and reinventing the very useful and versatile wheels we have in BIOVIA’s technology, but rather focus our attention on designing and refining the multiscale problem-solving scheme discussed above and applying our tools to address the problems. We use our collective team knowledge and expertise to best optimize the product of interest for our customers, as well as provide physical/chemical insight and rational-design workflows to enable our customers further engineer their product with a deeper (molecular level) insight. Our simulations’ results can be further passed along to more macroscale and cell level tools provided by other Dassault Systèmes brands, as indicated in Figure 3.
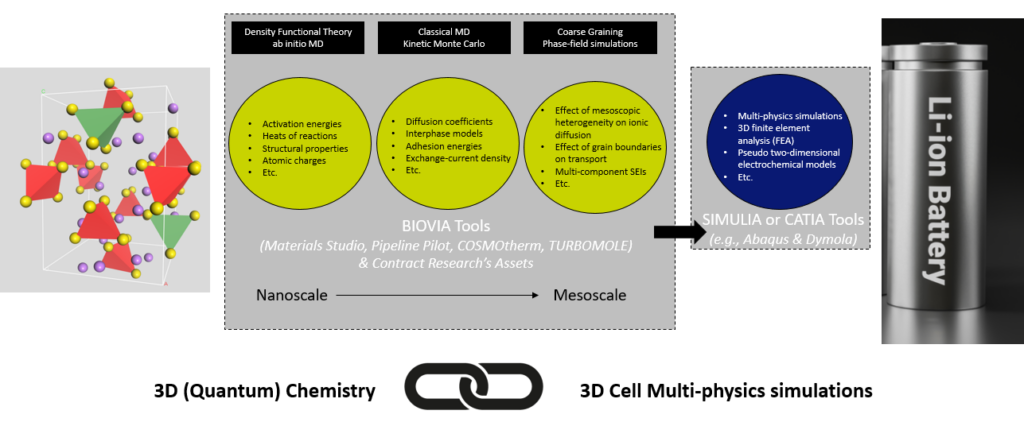
Figure 3: Some of the tools at our disposal to connect the quantum mechanical world of atoms and molecules to macroscale battery cells along with some of the properties we calculate and a subset of methods we use.
Modeling complex structures with a low barrier and handling large-scale simulations
The first thing that one experiences working with Materials Studio is the versatile visualizer tool that provides a great way to get more structural information about the material under study. We can also access tools that help us create compounds with many components. For example, once we have different types of solvent molecules and mixed Li salts, we can build the electrolyte “soup” using the Amorphous Cell module. This module adds molecules into a 3D box, one segment at a time, taking into account interactions with previous segments (a forcefield has to be assigned to calculate such interactions). The density is gradually built up towards a pre-specified target. This algorithm can yield disordered systems featuring realistic equilibrium conformations. Once such a structure is made, one can add other components as needed (e.g., adding more Li+ to reflect an increase/decrease in its concentration upon varying the charge state of the battery) using the Sorption module. The module can utilize different types of Monte Carlo simulations, e.g., Metropolis and the configurational bias methods. After building the structure (and equilibrating it through MD calculations) one has access to a variety of tools to analyze the structural properties of the compound, e.g., we can easily generate the Connolly surfaces, making us able to, e.g., quantify and visualize (in 3D or 2D) the “free” volume for Li+ cations to diffuse inside the electrolyte or towards/away from the electrodes. The analysis tools that are accessible through different modules, e.g., Forcite can generate great insight into the structural (and transport) properties of the system. To name a few, density fields, radial distribution functions, and mean square displacement for different species can be generated. Using the latter, one can readily access the diffusion coefficients (and thus conductivities and transference numbers) for multiple species (e.g., Li+ or anions).
Here is another example of complex structure generation: Materials Studio provides great functionality in working with polymer (solid-state) electrolytes. One can easily build polymers with arbitrary chain lengths and molecular weights starting from their monomer building blocks. Using the Conformers module, one has access to a variety of methods (e.g., random sampling and Boltzmann jump) to explore the lowest energy conformations of a polymer, which can become non-trivial at larger lengths. One can then mix a variety of polymers, solvents, and Li-salts, using the previously-discussed Amorphous Cell builder, to render realistic models of a gel-polymer solid-state electrolyte.
One common practice for our researchers in the Contract Research department is to use such useful functionalities to create many candidate systems and benchmark them against available experimental data, or benchmark a higher-level (more computationally expensive) method against a less accurate (less-expensive) one, and determine which level of theory is “good enough.” Hence, once dealing with complex and large-scale systems, our experts can achieve atomistic modeling precision, while still having a problem that is inexpensive enough to solve in a finite time and with finite resources. Another example of the expert input we can add is how to approximate formulations and complex stoichiometry of some compounds and formulations with simpler ones, and what computational parameters to use for simulating them to provide the best accuracy in the shortest amount of time and with minimal computational resources. These steps can become crucial for our customers after we provide them with initial workflow, and they try to further use this methodology to engineer their products: we can make such future studies come to fruition, say, in one month as opposed to one year, by providing an optimal, chemically/physically realistic methodology and workflow.
Insights into solid-electrolyte interphase (SEI) formation and evolution
SEI is a thin layer of material that forms under the reducing potential of the anode in the interface with the electrolyte material (see Figure 4). Although it occupies just a tiny portion of the battery’s volume, it has a great influence on the most important battery properties including the safety, efficiency, and durability of the Li-ion batteries. For example, the ionic conductivity of the SEI can determine the effective Li+ conductivity through the cell, while its mechanical properties can affect the Li-dendrite formation or the cell breakdown under cycling, thermal or mechanical strain.
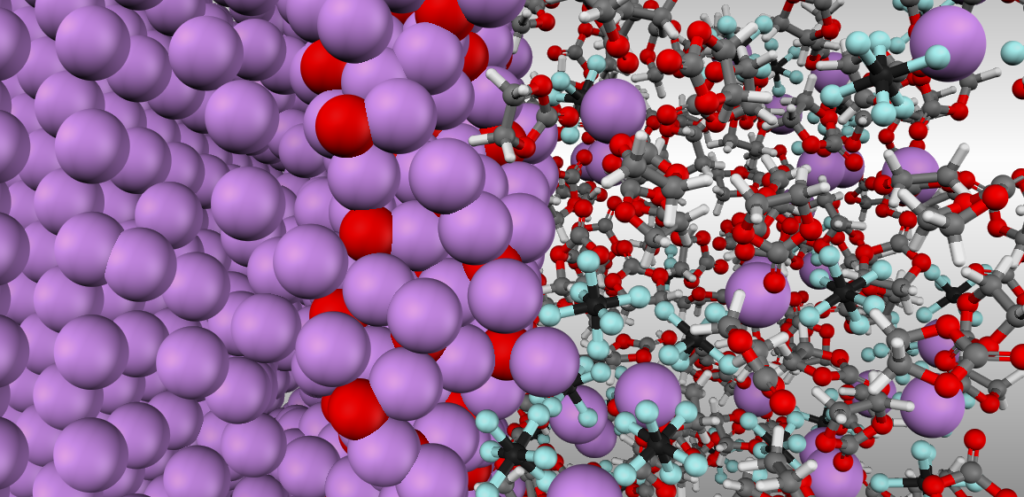
Figure 4: An atomistic simulation of an anode-electrolyte interface. On the left: a Li-metal anode. In the middle: Li2O interphase. On the right: an organic carbonate-based solvent with solvated LiPF6 salt complexes.
SEI formation is also a perfect example of how battery design effort has to be multiscale. In the first place, what generates the SEI is the interaction between the electrolyte molecules and the anode. To describe such (reactive) interactions, we need to calculate the heat of reactions and barriers using a quantum chemistry level method (e.g., DMOL3). We can then feed such data to the Reaction Finder module in Materials Studio, which once incorporated into a Monte Carlo-molecular dynamics (MC-MD) hybrid method can be used to simulate the formation of the SEI. Using the Monte Carlo simulations allows us to work with reactive cycles (corresponding to chemical reaction events some of which are rare ones) which significantly increases the timescale of the simulation. Additionally, the classical MD part of the algorithm significantly increases the length scale.
Being able to use a first-principles approach to grow SEI, we can, for instance, explore the role of electrolyte additives in stabilizing the battery durability through changes in the structure and properties of the SEI. After growing an SEI structure, we can afford to perform some large-scale quantum mechanical level calculations (e.g., using DFTB+, DMOL3, or CASTEP) to explore the density of states/band structure of the system and thus shed light on the electrical conductivity of the interface. We can further use classical MD calculations to derive the transport and mechanical properties of the interface (as a function of temperature and the charge state of the battery), providing great insight into the performance and durability of the cell. Having a model of the SEI also enables us to calculate the exchange current density.
Investigating the device integration capability and stability of the electrolyte
All-solid-state batteries have recently attracted great attention both in the academic and industrial circles. The promise of solid-state electrolytes (SSEs), as opposed to liquid-state organic-based electrolytes, is higher energy density (per volume and weight), better safety, and (ideally) more durability (a result of fewer reactions between the electrolyte and the electrodes). Nevertheless, in practice, the integration of SSEs into batteries has been challenging due to a lack of at least one factor among the list of device integrability, electrolyte stability, and high conductivity. Our computational toolkit at BIOVIA allows us to investigate and improve such properties using our virtual experiments and the chemical insight learned from them. For example, we can calculate the adhesion energy between a specific SSE formulation and the electrodes, and make this a factor in the rational design of the SSE material. Also, we can use quantum mechanical calculations to calculate the activation energies and heats of reactions that can, in principle, lead to the degradation of the SSE, and accordingly assign stability scores to the possible candidates in a high-throughput screening quest. Finally, we can calculate the hybrids of different components mixed in mesoscale (e.g., polymer and oxide elements), and calculate a range of conductivities for grains and grain boundaries in such composites. We can then create physical models that assign an overall conductivity score to such hybrids, taking various conduction channels and pathways into account.
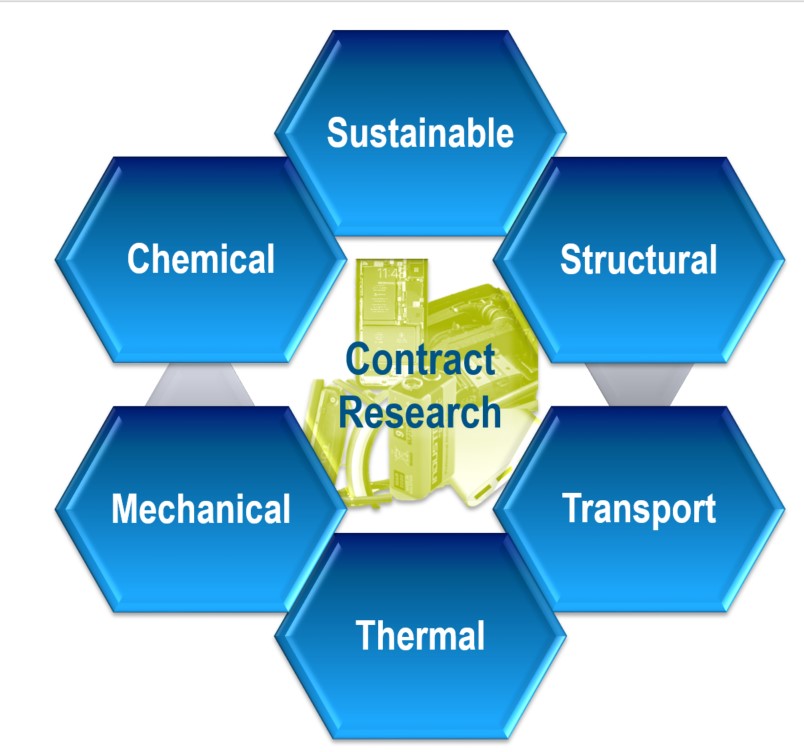
Figure 5: Some of the services and materials properties we deliver to our customers at Contract Research
A (very) complex problem, powerful proprietary tools, and experts that can deliver results
As briefly touched upon above, the atomistic battery design effort is, in principle, an extremely complex problem, stemming from various interacting components, electro-degradation of such components, and many side reactions. To literally expect to model the entire battery cell using atomistic modeling is nowhere close to feasible. Nevertheless, there is good news. Here at Dassault Systèmes, and the BIOVIA brand, we have developed versatile tools that can be applied to the types of problems that are of tremendous importance in battery design. Using our multidisciplinary expertise in the Contract Research department, we have developed proprietary Advanced Technology Capability (ATC) on top of the BIOVIA brand software. These proprietary ATCs encompass scientific algorithms (beyond what is in our software tool), content, validated models, and workflows that our customers will have access to when they engage with us in a collaborative project. Armed with BIOVIA’s software and our ATCs, we can embark on a journey that we can call “Solving a big problem by solving many smaller (multiscale) problem pieces.” Using numerical simulated experiments, we can verify whether such smaller problems can be later reassembled and fed into larger-scale simulations to create experimentally relevant observables or not. We have a workflow to suggest chemically/physically intuitive hypotheses linking the world of atoms and molecules to macroscopic phenomena. In a self-consistent manner, we revisit and readjust such hypotheses by comparing the results of our multiscale calculations with available experimental data. Once we have a working model, we will use our experience in atomistic modeling, to provide solutions to make a battery optimized for a specific use case and/or improve a special functionality or component in the battery. We are here to fill the knowledge gap between versatile BIOVIA’s (and Dassault Systèmes) tools and the real-world outcomes our customers’ desire. We are the experts who bring atomistic modeling and domain-specific data science to address grand challenges in battery innovation.