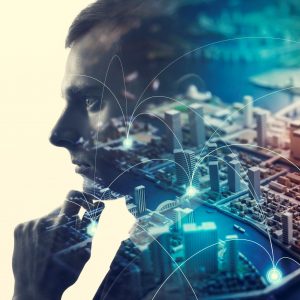
The daily headline today might as well be supply chain risk, for all the uncertainty and disruption companies face across their interlinked supply chains. AI may be the extra layer of smarts you need to keep ahead of—or more realistically, stay less far behind—the risk curve.
In supply chain, risk is always present. On the demand side, for example, even when times are peachy, no one can really know with a high degree of certainty what millions of consumers or business customers are going to need or do. AI systems can help demystify that demand—crunching through historical sales data, previous orders for clusters of products with similar attributes, and ongoing streams of point-of-sale data and social-media influencer sentiment.
In normal times—whatever that means anymore—machine learning can sift through that data to unlock patterns, trends and takeaways that it would be difficult for a supply chain planning team to uncover—and probably something they couldn’t get to amid the flurry of daily firefighting and regular chores. In disruptive times, giving a lightning-fast machine learning mind a seat at the table is even more vital to cope with the roller coaster ups and downs.
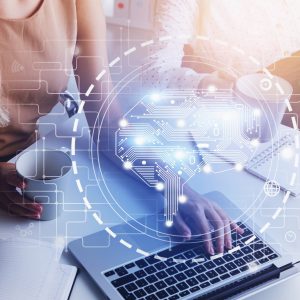
These machine learning algorithms are now embedded in advanced supply chain planning and optimization solutions. It’s a natural fit: SCP&O apps already bring to bear advanced statistical analysis and probability simulations in the way they run supply chain and logistics scenarios for current products, as well as to anticipate volumes for new product introductions and during promotional surges or unusual circumstances. Machine learning algorithms and analytics take that capability a step further to more deeply explore and visualize the data.
On the supply side, one monumental AI use could be to model from scratch entirely new chains during times of upheaval. It might do this by scraping global web data, then “learning” about and analyzing potential partners based on selection criteria such as their production locations, outsource competencies, on-time-in-full (OTIF) rates, ISO standards compliance, transit times from the nodes, capacity constraints, costs, sustainability reputation, and so forth.
Companies could benefit mightily by being able to run this exercise when times get tough in their supply chain. They could even do it on an ongoing basis, to continuously evaluate mix-and-match supply chain options that could either improve their current operations or be kept on the back burner for activation during periods of disruption and excessive risk.
While this kind of AI capability may still be a medium- to long-term wish-for, the data it needs to reach its conclusions is here now. Many companies globally are probably sorting through lots of this data at the moment, balancing the investment in their current supply chains with a series of alternatives—perhaps more distributed “mini” chains that offer multiple points of redundancy in times of need. AI, delivered via cloud-based SCP&O and business intelligence apps, could be a “human helper” for this most complex of supply chain risk management tasks.