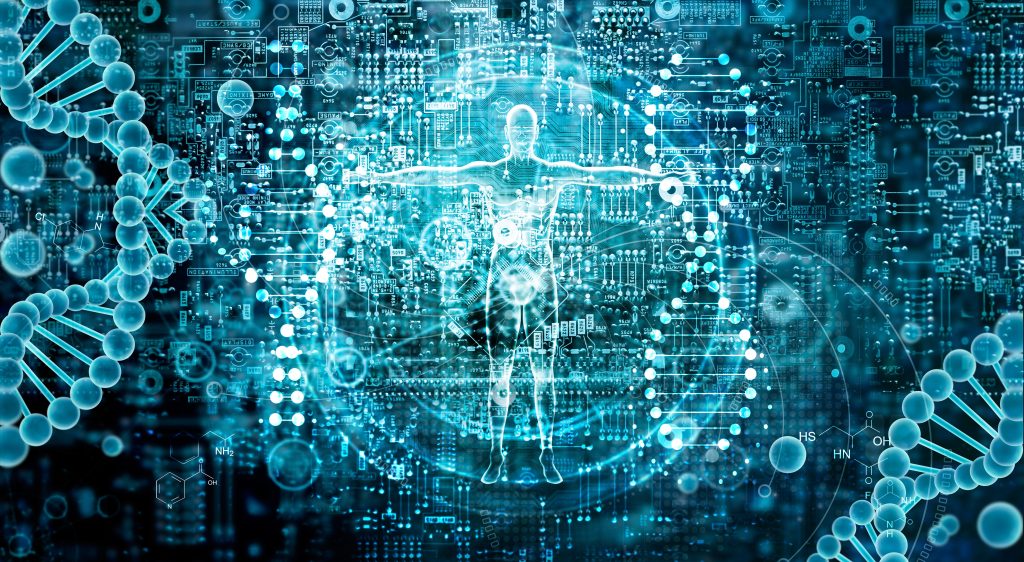
The FDA has already authorized AI/machine-learning-based medical devices that bring the Internet of Things (IoT) to the connected patient and deliver personalized healthcare—one for detecting diabetic retinopathy, another to alert for potential strokes. Now the agency is increasing its efforts to help bring Software as a Medical Device (SaMD) products to market, via a regulatory framework that will allow intelligent algorithms to learn and adapt based on real-world feedback, while at the same time ensuring device safety and effectiveness.
The thorny issue to be addressed is that, each time a device maker modifies a traditional SaMD product, it must submit documents that demonstrate the continued safety and effectiveness of the device after the changes. The wrinkle with AI and machine learning is that the device, over time, will operate differently on its own, based on what it learns in ongoing, real-world use.
That’s the conundrum the new framework seeks to tackle—how to safely let the software learn and adapt, to improve performance through the entire device lifecycle. The FDA has previously authorized AI and machine learning technologies that use “locked” algorithms, which don’t learn throughout use, but are modified and retrained by the manufacturer at intervals.
Continuous learning devices don’t require these manufacturer-induced changes to reflect new learnings or updates that enhance their operation—they learn from new user data themselves. That’s the driver for the FDA discussion paper issued on April 2 of this year, “Proposed Regulatory Framework for Modifications to Artificial Intelligence/Machine Learning (AL/ML)–Based Software As a Medical Device (SaMD).”
“The traditional paradigm of medical device regulation was not designed for adaptive AI/ML technologies, which have the potential to adapt and optimize device performance in real time to continuously improve healthcare for patients,” the paper states. “The highly iterative, autonomous, and adaptive nature of these tools requires a new, total product lifecycle (TPLC) regulatory approach that facilitates a rapid cycle of product improvement, and allows these devices to continuously improve by providing effective safeguards.”
The FDA is trying to balance regulatory oversight—keeping patient safety paramount—with continuous improvement of AI/ML device performance. It wants to establish good machine learning practices (GMLP), similar to current good manufacturing practices (CGMP). Premarket submissions for AI/ML devices must not only demonstrate reasonable assurance of safety and effectiveness, but continuous management of patient risks throughout the lifecycle. Device manufacturers must incorporate a risk management approach to algorithm changes. And the FDA will insist on transparency into real-world performance monitoring of the AI/ML device.
The regulatory body has its sights set squarely on the promise of AI/ML in healthcare. “I can envision a world where, one day, artificial intelligence can help detect and treat challenging health problems, for example by recognizing the signs of disease well in advance of what we can do today,” said outgoing FDA Commissioner Scott Gottlieb, M.D., in his April 2 statement. “These tools can provide more time for intervention, identifying effective therapies and ultimately saving lives.”