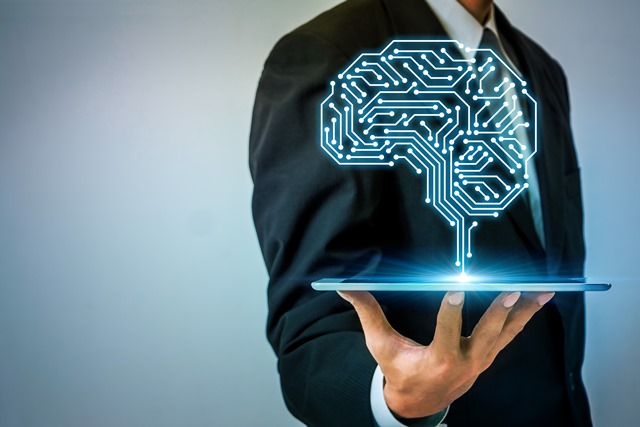
AI and machine learning are the new “big data.” Business executives are being told that if they don’t adopt the latest artificial intelligence tools, their companies are going to be disrupted, left to struggle and perish in the dustbin of the digital laggards.
Except that AI and machine learning are not “the latest” and without close scrutiny and thorough understanding may not even be “the greatest.” The best place to start understanding better what’s really new and promising and what to do about it is to briefly review their history.
The computer scientists giving birth to “Artificial Intelligence” in the mid-1950s were confident of the arrival in the near future of machines capable of human-level understanding and reasoning, as all intelligence—they argued—can be reduced to manipulating symbols and the mathematical formulas computers were so good at processing. But it didn’t work as advertised—it turned out that intelligence involves much more than defining concepts with logical rules. Instead of intelligent machines we got an “AI winter.”
An alternative approach to computerizing cognitive abilities was also born in the 1950s. It was called “machine learning,” a decidedly less sexy and attention-grabbing name. While the “artificial intelligence” approach was related to symbolic logic, a branch of mathematics, the “machine learning” approach was related to statistics.
There was another important distinction between the two: The “artificial intelligence” approach was part of the dominant computer science practice of a programmer telling the computer what to do by coding a program written in a specific programming language. The “machine learning” approach relied on statistical procedures that found patterns in the data or classified the data into different buckets, allowing the computer to “learn” (e.g., optimize the performance—accuracy—of a certain task) and “predict” (e.g., classify) the nature of new data that is fed to it.
For traditional programming, data was what the program processed and the output of that processing. With machine learning, the data itself defines what to do next. Over the years, machine learning has been applied successfully to problems such as spam filtering, hand-writing recognition, machine translation, fraud detection, and product recommendations.
Many successful Web-native companies such as Google, Amazon and Netflix, have built their fortunes with the help of clever machine learning algorithms. The real-world experiences of these companies have proved how successful machine learning can be in using lots of data from variety of sources to predict consumer behavior. Using lots and lots of data (or “big data”) makes predictive models more robust and predictions more accurate.
The recent excitement (and much hype) about AI is due to rapid advances in “deep learning,” a variant of machine learning largely based on the concept of artificial neural networks. Deep learning moves vast amounts of data through many layers of hardware and software (the artificial neural network), each layer coming up with its own representation of the data and passing what it “learned” to the next layer. A number of developments have come together to make deep neural networks very successful over the last five years: Better algorithms, big data, and increased computing power, specifically in the form Graphics Processing Units (GPU) which process data in parallel, thus cutting down on the time required to train the computer.
Deep neural networks have broadened the range of practical applications machines can learn and perform. They particularly perform well in narrow cognitive tasks such as object identification, machine translation, and speech recognition. Their success had also influenced the larger field of machine learning and motivated many enterprises to experiment with machine learning tools to discover new insights in the data they collect.
So today’s artificial intelligence and machine learning and deep learning approaches are simply new variants of age-old attempts to apply computers to an increasingly wider range of tasks. They are making a positive impact on enterprises but not in the form of intelligence equal or superior to human intelligence, but as practical business applications. They are promising but business executives must be aware of their specific potential pitfalls and be prepared for the difficulties of adopting new tools and getting the organization used to new practices.
The first thing to do is to identify the specific activities that could benefit from the predictive power of modern machine learning tools. Look for repeatable problems, for where it is easy for humans to miss a slight but significant variation in the flow of data that a machine learning tool would pick up, for opportunities to demonstrate immediate impact and a clear ROI.
Data—and its quantity and quality—plays a major role in ensuring a significant return on investment. This would be easier for enterprises who have already embarked on a digital transformation journey and have paid close attention in recent years to what data they collect (and what they need to collect) and to ensuring the data represents valid, consistent and “clean” real-life observations.
Another set of important considerations lies in the fundamental differences between the familiar—developing traditional software—and the new—developing and managing AI applications. Debugging is harder because it’s difficult to isolate a bug in the machine learning program. Unlike traditional software, when you change anything in a machine learning program, you end up changing everything. Most important, the trove of tools and tested processes that have been accumulated throughout the years for software development does not exist for modern machine learning. Learning from the experience of others and staying up-to-date regarding the latest developments in the practice of machine learning is crucial.
This expertise already exists, in many organizations, in the analytics and data science team so that’s a natural place to go to for incubating and driving early AI initiatives. Depending on the organization, it could be useful to select a senior member of that team to act as a Chief AI Executive, with enterprise-wide responsibilities for introducing modern machine learning methods.
As always, people are the most important element in ensuring the success of the introduction of new tools and practices. Not only the people managing it, but also the people on the receiving end, the employees that must adjust the way they work and understand the promised benefits of the new tools. Given the bad rap AI sometimes get in the press—and the ominous-sounding, human-replacing flavor of “artificial intelligence”—paying close attention to how it is introduced into the organization is probably even more important than it typically is with other new technologies. The emphasis should be on human “augmentation,” not “automation,” and the creation of new roles and responsibilities in the enterprise.
As we succeed in computerizing cognitive capabilities, computers will continue to augment humans, as they have done for more than sixty years. For enterprises, this means working smarter and finding new ways to thrive.
Editor’s Note: Industry leaders will be coming together this year to discuss the role of AI and Machine learning and what’s next to enable the industry renaissance. To learn more register here for the 3DEXPERIENCE FORUM 2019, taking place May 13-16 at Caesars Palace, Las Vegas.