In recent years, GEOVIA has leveraged the expansive portfolio of software and technology within Dassault Systèmes to bring new features and functionality to market.
One software package with tools beneficial to mining is SIMULIA Isight, a desktop solution for workflow automation, optimization and simulation. Geologists and mine planners can utilize this technology to validate block model estimates and production schedules. In this post we will look at how SIMULIA Isight can be applied by geologists within traditional block modelling workflows to optimize and validate estimation parameters.
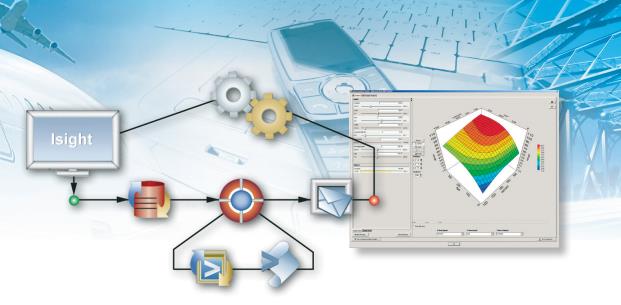
Two example scenarios of SIMULIA Isight working with GEOVIA Surpac are given below, a block size optimization and an estimation parameter optimization.
Example 1
This example illustrates a block size optimization scenario in SIMULIA Isight automated 120 Ordinary Kriging block model estimations in GEOVIA Surpac software, testing 120 different block size combinations in X and Y. It was hoped this analysis would help support existing reasoning and suspicions over the optimum block size by providing statistical evidence, from Kriging Variance (KV), Kriging Efficiency (KE) and Conditional Bias Slope (CBS).
SIMULIA Isight presents the results as a set of interactive tables and graphical charts, designed to help the user interpret the data and make informed decisions about which estimation yields the ‘best’ result. A summary of the results is presented below.
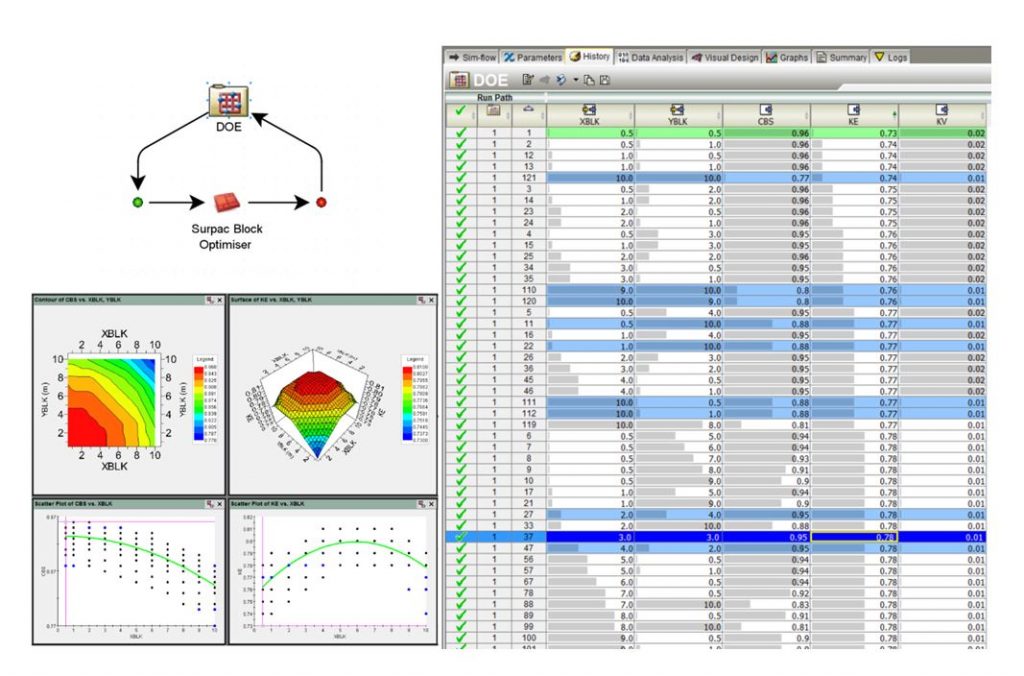
As seen above, Isight will use target values specified by the user to highlight a selection of the best cases. In this example, target values used were CBS = 1, KE = 1 and KV = 0.
From these best cases (light blue rows) the optimal block size can be determined, a decision which should take into account all the statistics presented and any supporting information that may exist. From the assessment of the results, a block size of 3m by 3m was selected for the X and Y parent block size.
While Surpac’s macro capabilities can help to streamline many processes, Isight can assess many more estimations in the hundreds, thousands and tens of thousands. The results can be sorted automatically on targeted criteria to easily identify the optimal or ‘best’ case.
Example 2
The second example, an estimation parameters optimization in SIMULIA Isight, will use the block size and data identified above to further optimize the associated estimation parameters, specifically:
- Minimum number of samples
- Maximum number of samples
- Maximum search distance
- Nugget value
- Sill
- Range
This results in 702 Ordinary Kriging estimations, with three different values assessed for each parameter. Once again, the statistics of Kriging Variance, Kriging Efficiency and Conditional Bias Slope, were used to evaluate the estimates, with the same target values of CBS = 1, KE = 1 and KV = 0. The results are presented below, with the selected case highlighted in blue, along with the final model in Surpac.
Advantages of using SIMULIA ISight
Task Automation
Isight is a useful tool for running a process, whether that is for a block model estimation or a production schedule.
It allows for multiple software applications, parameters and values to be automated to ascertain the optimum case, with respect to the target values and output parameters. It is far faster and more efficient than running the same number of estimations manually or with a macro.
Ultimately, the same diligence and data validation procedures should be applied to the final results presented by Isight, as with any standard block model estimation with Surpac.
Optimization
In addition to task automation discussed above, SIMULIA Isight can also perform optimization. This is the act of giving a range of values on multiple parameters and telling the software the desirable outcome. Isight will then optimize the parameters rather than testing all possible combinations and give you the best outcome.
It will also allow you to analyze whether improving 1 KPI adversely effects another KPI (this is known as Pareto Analysis). Sometimes, the optimal result for one parameter can be damaging for another and a mid-result would be better for all.
Simulation
Instead of inputting a range of variables, we instead input a distribution. This lets the software know that some outcomes are more common than others and as such this should be given more weight. Using simulation, we can measure the probability of an outcome.
If you are interested in SIMULIA Isight for optimizing part of your mining process or would like to discuss the possibilities, please email GEOVIA.Info@3ds.com where your query will be routed either to myself for the Europe or Middle East region, or one of my colleagues if you are based in a different part of the world.