We are seeing great advances in AI-driven design of novel therapeutics, but does that mean the medicinal chemist will become obsolete? Well, as it turns out, AI is good at many things, but a chemist with years of experience in a therapeutic area seems to know things that aren’t easily captured by AI. Can we somehow capture the chemist’s ‘intuition’ to make the AI better?
BIOVIA Generative Therapeutics Design (GTD) automates the process of designing molecules that are expected to have a specific set of properties. GTD accomplishes this task by evolving molecules through successive generations of changes, until the best-so-far structures are identified, based on the predictions of the relevant machine learning models. Recently, I was using GTD to discover new molecules with a simple, but elusive, specific set of properties. My goal was to identify structures that were in the training set for my models but meaningfully different from what was already known and still active on my target. In such cases, generative, machine learning-based applications often suggest molecules which meet the criteria and pass all the structural rules, but are still a bit ‘ugly’ from a chemist’s perspective.
At the outset, I had run a few experiments looking for molecules that would meet my Target Product Profile (TPP). In this case, these molecules should help improve a Dopamine D2 receptor modulator model by finding examples in areas of chemical space, which were underrepresented in the training data, but meet any TPP. Looking at the results of these experiments, I saw substructures that I would rather not have. (Fig 1.) Now, I could go tweak my Filter settings to be more strict about removing ‘Bad Substructures’ or sticking to ‘Known Fragments’. But, since this task can be time consuming, I decided to use a feature of GTD that allows users to build a model of their opinion about the molecules by ‘rating’ them and learning a model of those ratings.
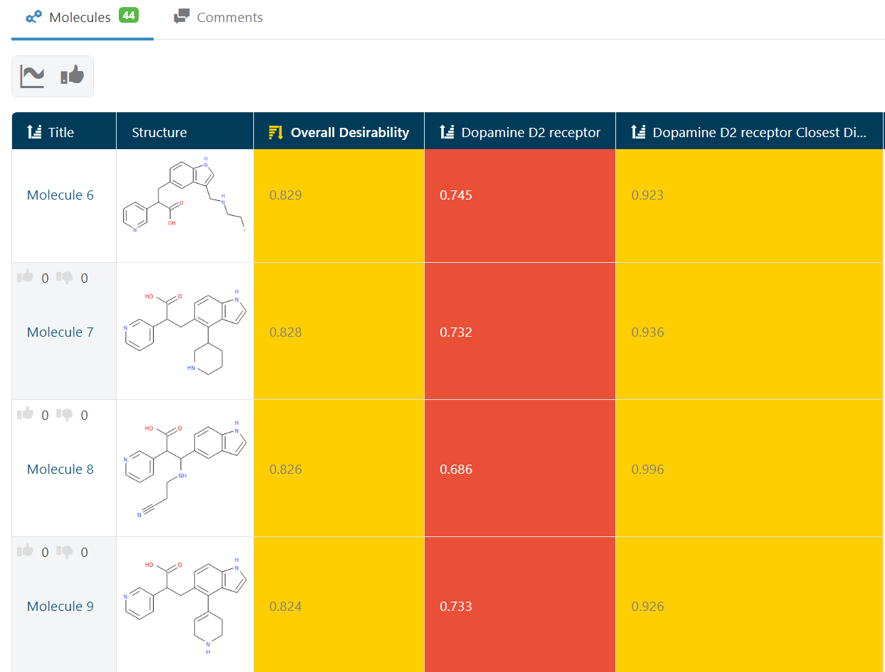
The first step was to use the Molecule Review interface in GTD. This interface can be considered as the “Tinder for molecules” where each structure is quickly seen with some key stats and Liked or Disliked by the user with a single key stroke. (Fig 2) Giving a rating automatically brings up the next candidate. In a matter of minutes, I had 144 ratings stored. (Fig 3)
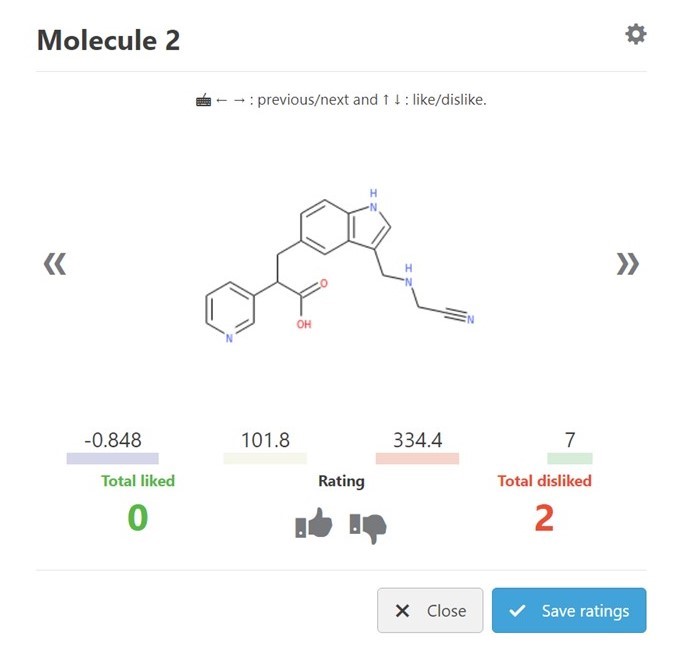
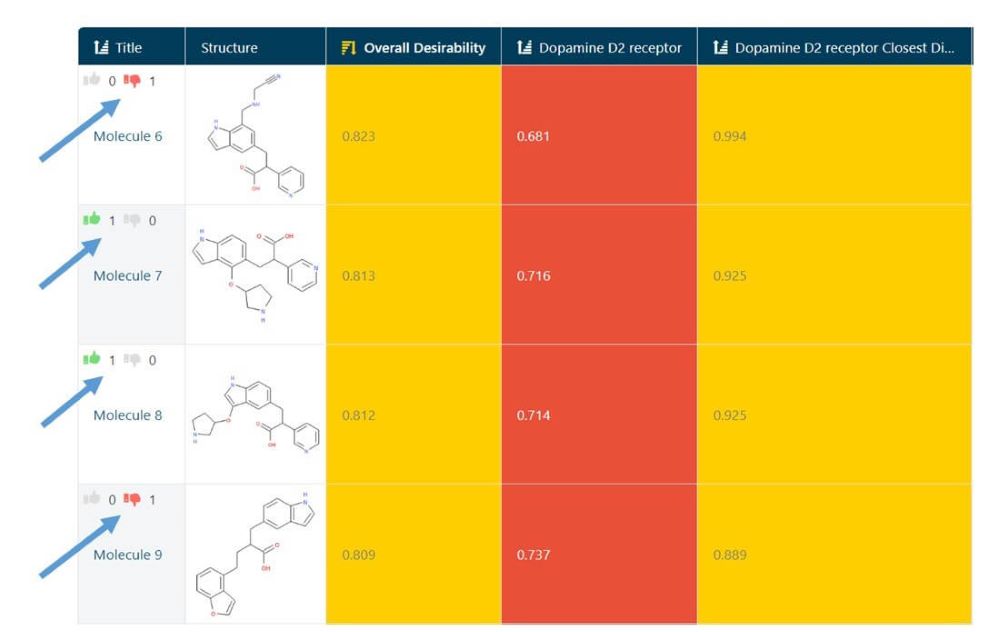
Next, in the Study interface, I downloaded an SD file of all molecules that had been rated in the context of this Study. (Fig 4) This step allows me to combine ratings from all users into a single model, make a model of just my ratings, or apply any other more complex thresholding. In this case, I used the file as is, since I only wanted to model my ratings.
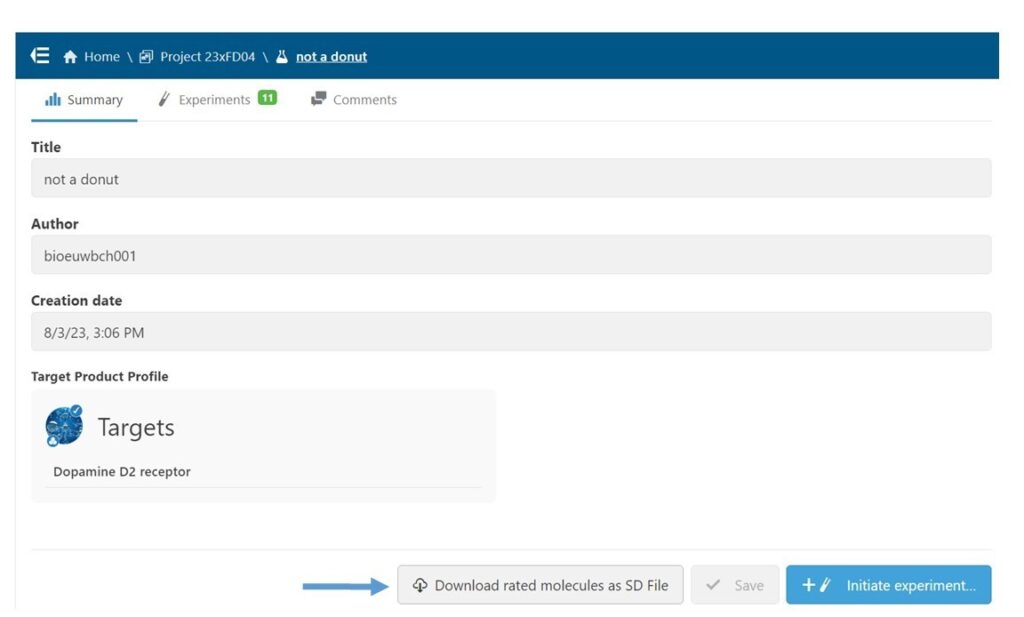
To build the model, I dropped the file into the 3DDrive and dragged it into the Build Model Input box in GTD’s model builder. (Fig 5) In this case, the various Response Properties predefined in the file were all equivalent, so I chose myRating. Setting the Positive Category to ‘1’, aligns the ‘good’ molecules category with my ‘Like’ ratings. (Setting it to -1 would model what I didn’t like.) Building the model took just a few seconds, and then I was ready to use it.
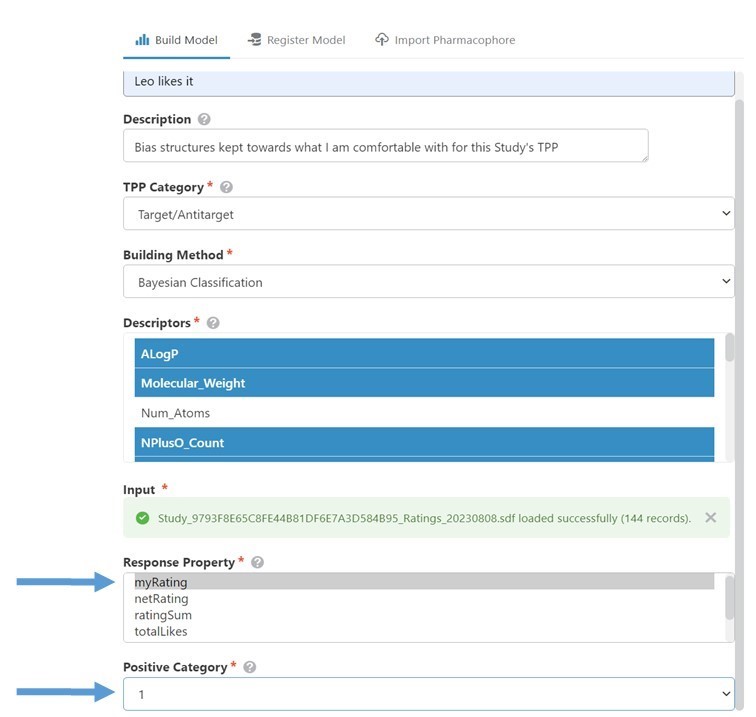
Since each Study has a defined TPP, I built a new one with my new model as a Target along with the Dopamine D2 receptor modulator model I had been using before. I added an Experiment and imported the settings from the previous runs. The last step was to set the Desirability curve for the model of my likes (usefully named Leo_likes_it). In the settings, you can see how well the learner could build a model to separate the molecules I liked from those I disliked. (Fig 6) Then, I just pressed ‘Run’. Reviewing the output molecules from this experiment showed a huge decrease in the prevalence of the substructures that had been bothersome in the earlier runs. Success!
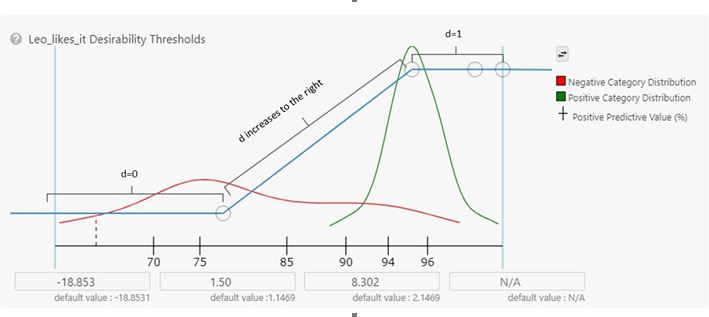
Naturally, I could rate more molecules and create a better model, collaborate with my team to build a consensus model, build models specific to a project or applicable across the organization. All it takes is to get people to use GTD’s rating system and we have achieved the best of both worlds: human-in-the-loop AI!
To learn more about BIOVIA Generative Therapeutics Design, download the datasheet.