With so many different parameters to vary, a full design space exploration using simulation might be prohibitively time-consuming. This means that engineers often have to resort to trial and error to find a welding process that produces parts of sufficient quality. Machine learning, using deep learning neural networks trained on simulation results, can significantly speed up weld optimization.
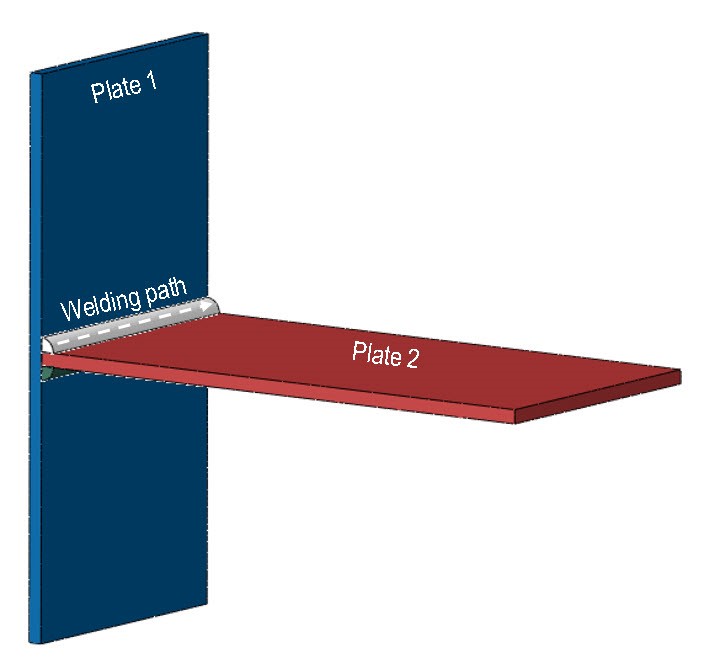
The image above shows an example of a simple weld optimization, using two plates of nickel-based superalloy IN625 measuring 200 × 100 × 5 mm. The welding simulation utilizes Abaqus software for sequentially coupled thermo-mechanical analysis, employing the Additive Manufacturing (AM) process simulation interface for flexible definition of welding paths, parameters, and heat flux distribution. A dataset of 80 simulations varying welding power (400-600 kW), speed (0.5-1.0 m/s), and torch angle (30-90 deg) is generated. 64 of these are used to train a neural network-based AI model, with the remaining 16 used to test it.
The trained neural network acts a surrogate model of the welding process. Once trained, it can calculate the 3D deformation and residual stress almost instantly for new parameters, representing a speed-up of over 100,000% compared to simulation alone. The model can generate many different types of result, including melt pool shape, temperature distribution, distortion and residual stress. The surrogate model shows excellent accuracy, with around 0.1% error in maximum deformation compared to the reference simulation.
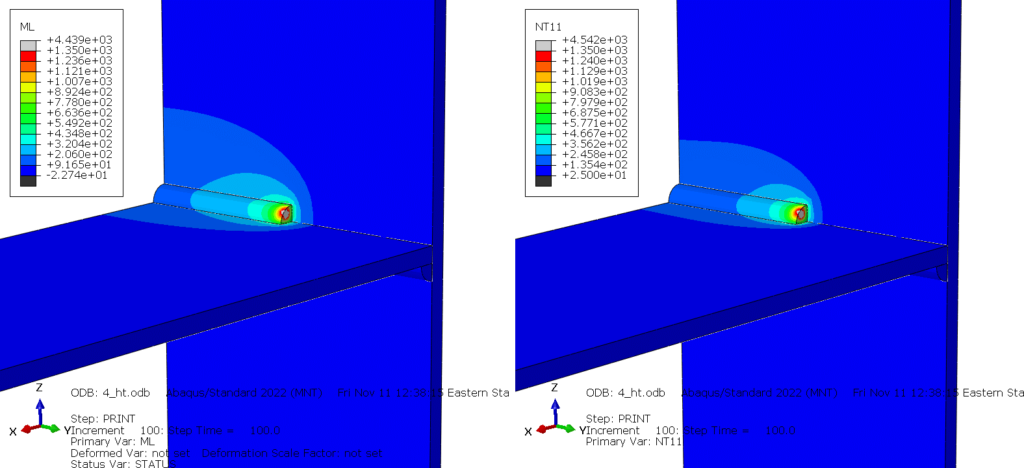
The results from the surrogate model can also be used as an input for further simulations. The temperature distribution from a deep learning model for instance can be used for a thermal stress FEA simulation. In this way, machine learning and AI becomes a powerful tool for multi-scale, multi-physics analysis.
Conclusion
Using AI and machine learning to support simulation, engineers can not only analyze the integrity of thee weld, but optimize the properties of the weld quickly and efficiently. This allows engineers to improve the integrity of the joint and help ensure that safety and quality targets are met.
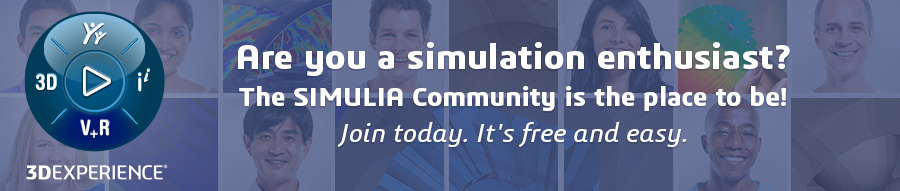
Interested in the latest in simulation? Looking for advice and best practices? Want to discuss simulation with fellow users and Dassault Systèmes experts? The SIMULIA Community is the place to find the latest resources for SIMULIA software and to collaborate with other users. The key that unlocks the door of innovative thinking and knowledge building, the SIMULIA Community provides you with the tools you need to expand your knowledge, whenever and wherever.