
Alzheimer’s disease is a neurodegenerative disorder that accounts for most dementia diagnoses. People with this disease undergo cognitive impairment, memory loss, and behavioral problems, which bring them physical and mental difficulties in their daily lives. The pathological features of Alzheimer’s disease are neuronal cell death, neuroinflammation, synaptic loss, and the accumulation of two definitive pathological lesions: Amyloid plaques and Neurofibrillary Tangles [1]. These two lesions are caused by the deposition and aggregation of amyloid β (Aβ) and τ protein, respectively, which play critical roles in Alzheimer’s disease neuropathology. Consequently, these two markers are pharmaceutical trial’s most common therapeutic targets.
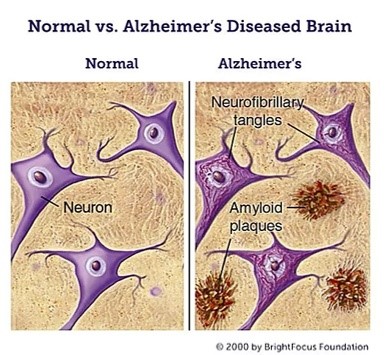
Alzheimer’s disease has been investigated for several decades, but a definitive understanding of its pathogenesis and an effective cure has yet to be discovered. Various hypotheses have been proposed to explain this pathology: Amyloid hypothesis, Tau hypothesis, Calcium hypothesis, Inflammation hypothesis, Diabetes hypothesis and Mitochondria hypothesis. Using Living Map, we intend to build a Boolean Network model that encompasses these various hypotheses, which may help find a treatment for this disease.
Systems Biology modeling with Boolean Networks
Though Partial Differential Equations are one of the most common formalisms to describe real-life systems (such as the fall of an apple, the movement of planets or how planes fly), their use is limited when describing biological systems. Using differential equations for biological systems would require precise knowledge of numerical parameters that lie out of direct measurement reach. Other formalisms can be considered to circumvent this issue such as Boolean Networks, an approach used successfully in Systems Biology for the past twenty years.
In a Boolean Network, the various entities involved in a system are described as nodes with a Boolean state (True when the entity is active and False otherwise). These nodes are connected by edges representing the node activation as an edge with an arrow and node inhibition as an edge with a blunt. Along with the usual logical operators (AND, OR, NOT), these edges encode the whole logic of the system. Its dynamic can then be simulated (by updating each entity state according to the Boolean rules of the network). Though they will not be as detailed as differential systems (also called quantitative simulation), the results of such a qualitative simulation can give an “idea” of the overall behavior of the system under consideration.
Navigating Systems Biology with a Living Map
BIOVIA Living Map is a service on the Dassault Systèmes 3DEXPERIENCE® Platform that provides automated knowledge access, modeling, and simulation capabilities for a given scientific context such as systems biology. The BIOVIA Living Map application currently leverages these capabilities to allow system biologists and mathematicians to design and simulate Boolean biological networks. It comes with a set of scientifically validated Boolean signaling pathways that scientists can use to modify existing experiments, create new experiments and test new hypotheses. A key feature of BIOVIA Living Map is its ability to automate entity and relation mapping to renowned cross-references, scientifically validating the designed model. We refer to the aggregation of a Living Map model such as a pathway and its cross-references as a knowledge map.
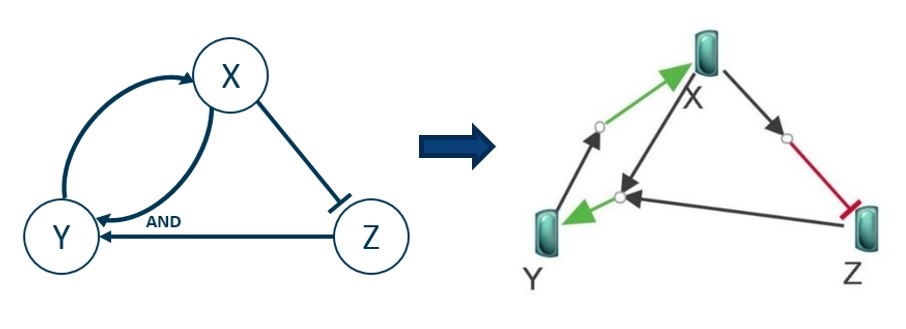
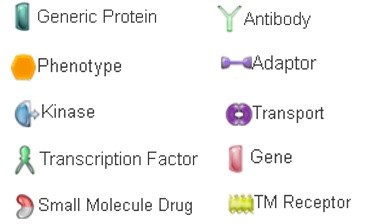
Examples of Alzheimer’s Disease Hypotheses Considered
As mentioned previously, though we do not yet have a clear understanding of Alzheimer’s disease, several hypotheses intend to explain its development. Hereafter, we detail some of the leading hypotheses reported in the literature.
Amyloid Hypothesis
One of the main mechanisms of Alzheimer’s disease pathology is amyloidopathy, the deposition of Amyloid-β(Aβ) that results in the formation of extracellular amyloid plaques in the brain. Amyloid plaques in the brain cause multiple pathological consequences, such as synaptic signaling disruption and cell death. ”Amyloid hypothesis”, suggested by Hardy and Higgins in 1992, is the most credible explanation of Alzheimer’s disease pathology so far [2]. Current research on this hypothesis focuses primarily on oligomeric Aβ and its role in Alzheimer’s disease as the key factor. [3]. Insoluble Aβ peptides are produced from amyloid precursor protein (APP), a transmembrane protein, through cleavage by β-secretase (or BACE-1) and γ-secretase, while normally APP is cleaved by β-secretase and γ-secretase producing soluble peptides (figure 4). Extracellular amyloid plaques formed from the aggregation of Aβ species can be removed by multiple mechanisms, such as microglial phagocytosis, transportation through the blood-brain barrier and degrading enzymes. In this manner, variations in normal Aβ formation, aggregation or clearance may result in severe amyloidopathy in Alzheimer’s disease [4, 5].
Tau Hypothesis
Along with amyloidopathy, tauopathy is one key mechanism of Alzheimer’s disease pathogenesis. Tauopathy is characterized by intracellular aggregation of microtubule-associated τ protein into NeuroFibrillary Tangles which are strongly toxic for neuronal cells. As presented in figure 4, τ protein can be first disassociated by phosphorylation at certain microtubule-binding residues, which impacts microtubule integration, thus causing defective axonal transport in neurons [4, 5].
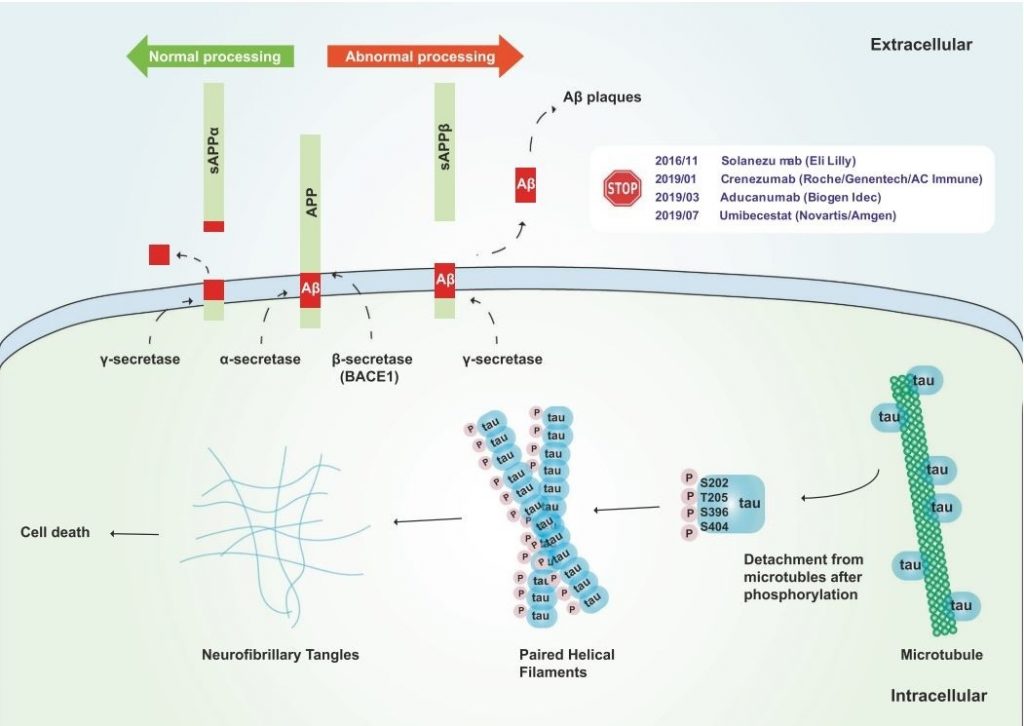
Calcium Hypothesis
In cells, especially neuronal cells, calcium is used as an essential mediator for numerous signaling pathways through its level fluctuation in the cytosol [6]. In other words, disruption of calcium homeostasis in neuronal cells may result in neuropathogenesis. Thus, the calcium hypothesis involves multiple calcium transporters in calcium-retaining cell organelles, such as the endoplasmic reticulum and mitochondria, calcium-binding signaling molecules and neurotransmitters whose release is controlled by calcium [7, 8, 9, 10].
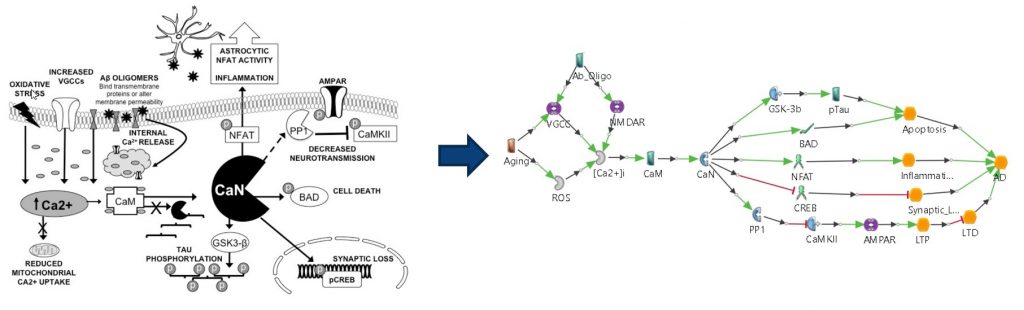
Inflammation Hypothesis
Inflammation has been suggested as a potent factor in inducing neuronal damage, and chronic inflammation seems to be linked with neurodegenerative diseases. Inflammation in the brain generally impacts microglia and astrocytes as well as neurons. Microglia and astrocytes also have abilities for extracellular Amyloidβ clearance [11, 12, 13, 14].
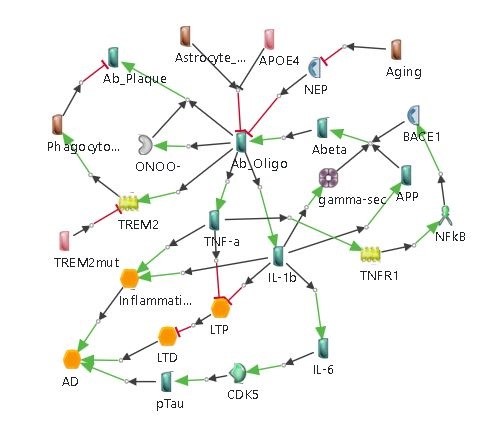
Diabetes Hypothesis
Diabetes has been shown to be associated with Alzheimer’s disease, and vice versa, suggesting that there is a common mechanism between the two conditions. Studies show insulin resistance as a causal factor of Alzheimer’s disease. The link between the insulin pathway and Alzheimer’s disease pathogenesis involves the Forkhead box class O (FoxO) transcription factor, which plays a role in glucose metabolism and oxidative stress response [15, 16, 17].
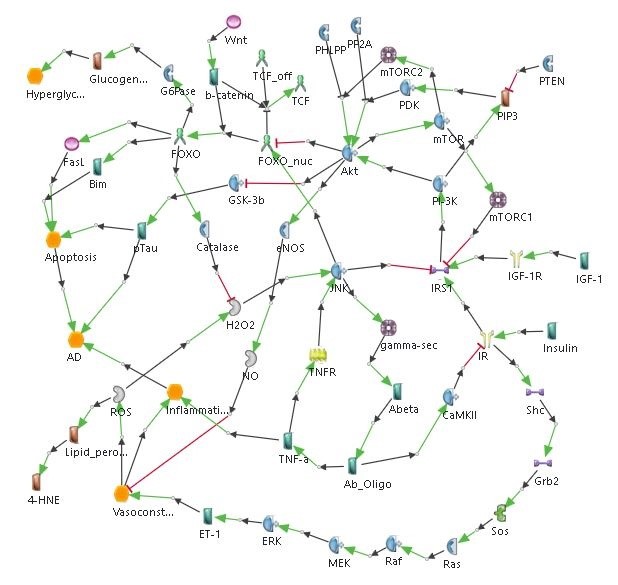
Mitochondrial Cascade Hypothesis
Mitochondria plays a significant role in the cell, managing oxidative stress, calcium homeostasis, apoptosis, etc. In Alzheimer’s disease, mitochondrial function is hugely reduced. As a result, the level of reactive oxygen species (ROS) increases, thus leading to the apoptotic process induction [18]. Mitophagy (autophagy of mitochondria) is observed to be decreased in Alzheimer’s disease, meaning that dysfunctional mitochondria are not properly removed causing further damage in the cell [19, 20].
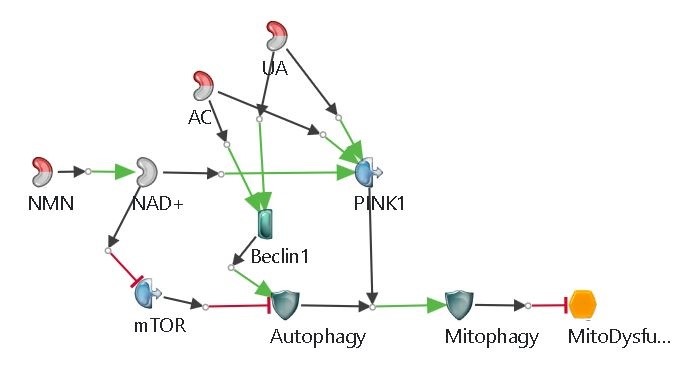
Merged Model and Results
We built twenty models of different potential mechanisms leading to Alzheimer’s disease. Using a proprietary algorithm, we assembled these pathways in a single merged model with almost 200 entities and 300 interactions (with as many relevant annotations as possible).
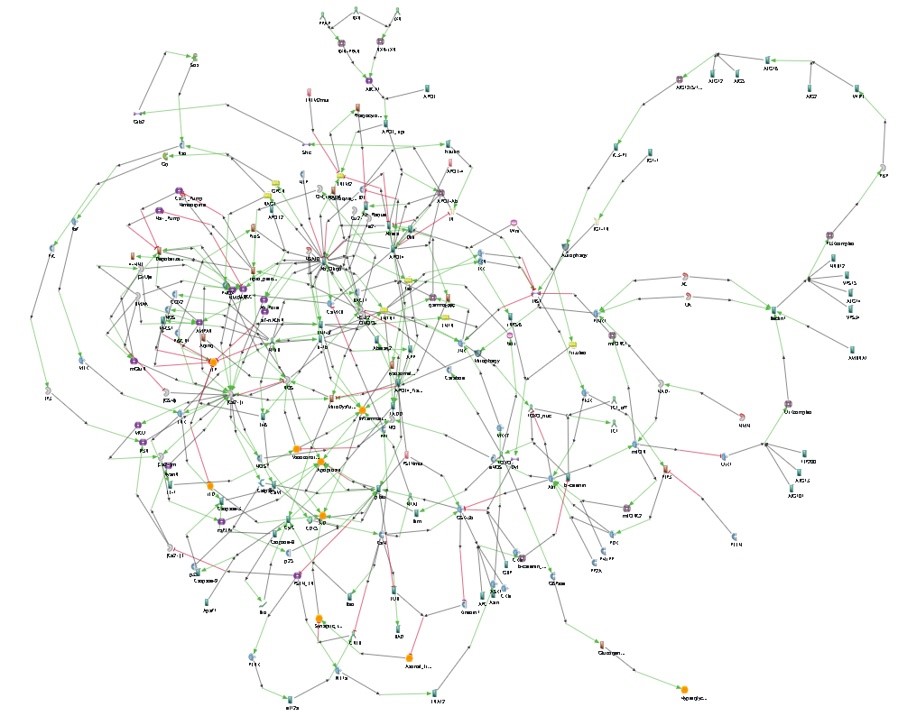
We then used Living Map to simulate the resulting pathway under various conditions. Preliminary results showed some unexpected behavior. For instance, according to the model, all aged patients would develop Alzheimer’s disease, which fortunately is not the case. A possible interpretation of these results is the merging of the various pathways may have been a too simplistic approach: the phenotypes causal structure is not properly described by the elementary pathways. Thus, changing the hierarchy of phenotypes in the merged model indeed led to better results.
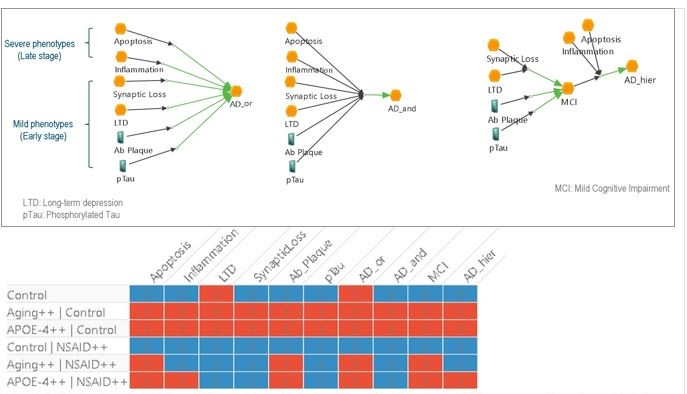
Conclusion and Future Works
Biologists often perceive systems biology modeling as a hurdle because they have to manually manage mathematical calculations of Boolean networks based on their hypotheses or by developing tools to do so. Either this leads biologists to be non-exhaustive in manual calculations that can be error-prone or it forces them to dedicate time or money to development work that is not their main field of competency.
The Living Map application relieves biologists from this burden by allowing them to build dynamic models representing a virtual twin of the diseased cell that mimics the disease behavior and shows results that correspond to disease outcomes as seen in clinical trials. When the virtual simulations of the model give accurate results, a model can then be used for virtual experiments to test new hypotheses or repurpose drugs for example.
In this manner, we used the Living Map application to build a first dynamic model of a holistic virtual cell that mimics Alzheimer’s disease pathologies as documented in scientific publications.
Although significant efforts have been done in the public domain to build AD-related pathway databases [21, 22], it is the first time that a single platform provides a model repository with both modeling and simulation capabilities in a single web application. Avoiding the data transfer between different software, formats, and systems, saved us a lot of time. Thanks to our proprietary merging algorithm, Living Map leverages pre-existing knowledge by providing an accessible and controlled way to import and assemble cell-signaling pathways from various sources. So rather than starting ex nihilo, we could quickly grow our model by importing and merging published knowledge, leaving us with more time to investigate disease behavior through virtual experiments and unravel its drug mode of action. This same methodology was used to build our SARS-CoV-2 model to avoid fatal cytokine storm.
We need to continue calibrating this virtual cell so that the aggregated version accurately reproduces the expected outcomes as observed in clinical trials. This result can be achieved by utilizing the Dassault Systèmes 3DEXPERIENCE cloud to collaboratively test and verify biological facts (from the scientific literature) to validate various sub-models and their successive aggregation. Furthermore, Boolean logic may be over-simplistic since it allows only two states and limits our description of threshold mechanisms. For this reason, we consider adopting a multi-valued logic in Living Map to depict some progressive disease steps. To fully understand Alzheimer’s disease and its pathogenesis, we must continue reading and studying current literature. Science is always a work in progress and even theories change. As research continues and new evidence emerges, all new hypotheses need to be considered.
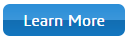
Acknowledgements
Special thanks to our former intern Yangji Choi (currently doing her PhD at Centre Hospitalier Universitaire Vaudois in Lausanne, Switzerland) who built the models and did the bulk of the work reported here.
On the recent amyloid controversy:
Since we conducted the work reported here, doubts have emerged about data manipulation and the veracity of some research based on the amyloid hypothesis [23]. To the best of our knowledge, the current controversy centers on a 56-petptide amyloid residue that we did not take into account in our pathway models. However, we do agree that the precise role of amyloid beta plaques in Alzheimer’s disease is not yet clearly understood and that further research is required (as evidenced with the troublesome FDA approval of aducanumab).
References
- D. M. Holtzman, J. C. Morris, and A. M. Goate. STATE OF THE ART REVIEW Alzheimer’s Disease : The Challenge of the Second Century. 3(77), 2011.
- D. Teplow, M. Yang, R. Roychaudhuri, E. Pang, T.-P. Huynh, M.-S. Chen, and S. Beroukhim. The Amyloid β-Protein and Alzheimer’s Disease. pages 1–85. Apr 2011.
- R. H. Swerdlow, J. M. Burns, and S. M. Khan. The Alzheimer’s disease mitochondrial cascade hypothesis: Progress and perspectives, 2014.
- P.-P. Liu, Y. Xie, X.-Y. Meng, and J.-S. Kang. History and progress of hypotheses and clinical trials for Alzheimer’s disease. Signal Transduct. Target. Ther., 4(1), dec 2019.
- K. B. Magalingam, A. Radhakrishnan, N. S. Ping, and N. Haleagrahara. Current Concepts of Neurodegenerative Mechanisms in Alzheimer’s Disease, BioMed Research International 2018.
- M. J. Berridge, M. D. Bootman, and P. Lipp. Intraoperative Visualization of Bilateral Thrombosis in the Posterior Inferior Cerebellar Artery Apparent in the Telovelomedullary Segment. Case Rep. Neurol. Med., 2014(October):1–4, 2014.
- M. J. Berridge. Calcium hypothesis of Alzheimer’s disease. Pflugers Arch. Eur. J. Physiol., 459(3):441–449, 2010.
- I. Bezprozvanny and M. P. Mattson. Neuronal Calcium Mishandling and the Pathogenesis of Alzheimer’s Disease. Contemp. Crit. Care, 7(2):1–11, 2009.
- L. C. Reese and G. Taglialatela. A Role for Calcineurin in Alzheimers Disease. Curr. Neuropharmacol., 9(4):685–692, 2011.
- C. Supnet and I. Bezprozvanny. Neuronal calcium signaling, mitochondrial dysfunction, and Alzheimer’s disease, 2010.
- M. T. Heneka, D. T. Golenbock, and E. Latz. Innate immunity in Alzheimer’s disease. Nat. Immunol., 16(3):229–236, 2015.
- J. W. Kinney, S. M. Bemiller, A. S. Murtishaw, A. M. Leisgang, A. M. Salazar, and B. T. Lamb. Inflammation as a central mechanism in Alzheimer’s disease. Alzheimer’s Dement. Transl. Res. Clin. Interv., 4:575–590, 2018.
- F. Liao, H. Yoon, and J. Kim. Apolipoprotein e metabolism and functions in brain and its role in Alzheimer’s disease. Curr. Opin. Lipidol., 28(1):60–67, 2017.
- W. YingWang, M. S. Tan, J.-T. Yu, and L. Tan. Role of pro-inflammatory cytokines released from microglia in Alzheimer’s disease. Ann. Transl. Med., 3(10):1–15, 2015.
- K. N. Manolopoulos, L. O. Klotz, P. Korsten, S. R. Bornstein, and A. Barthel. Linking Alzheimer’s disease to insulin resistance: The FoxO response to oxidative stress. Mol. Psychiatry, 15(11):1046–1052, 2010.
- C. O’Neill. PI3-kinase/Akt/mTOR signaling: Impaired on/off switches in aging, cognitive decline and Alzheimer’s disease. Exp. Gerontol., 48(7):647–653, 2013.
- P. Storz. Forkhead homeobox type O transcription factors in the responses to oxidative stress. Antioxidants Redox Signal., 14(4):593–605, 2011
- E. Fang, H. Y. Jun, and K. Palikaras. Mitophagy inhibits amyloid-β and τ pathology and reverses cognitive deficits in models of Alzheimer’s disease. Nat Neurosci., 176(3):139–148, 2019.
- E. F. Fang. Mitophagy and NAD+ inhibit Alzheimer disease, jun 2019.
- S. Lautrup, G. Lou, Y. Aman, H. Nilsen, J. Tao, and E. F. Fang. Microglial mitophagy mitigates neuroinflammation in Alzheimer’s disease. Neurochem. Int., 129, Oct 2019.
- S. Ogishima, S. Mizuno, M. Kikuchi, A. Miyashita, R. Kuwano, H. Tanaka, J. Nakaya. AlzPathway, an Updated Map of Curated Signaling Pathways: Towards Deciphering Alzheimer’s Disease Pathogenesis. Methods Mol Biol. 1303:423-432, 2016.
- D. Croft, G. O’Kelly, G. Wu G, R. Haw, M. Gillespie, L. Matthews, M. Caudy, P. Garapati, G. Gopinath, B. Jassal B, S. Jupe, I. Kalatskaya, S. Mahajan, B. May, N. Ndegwa, E. Schmidt, V. Shamovsky, C. Yung, E. Birney, H. Hermjakob, P. D’Eustachio, L. Stein. Reactome: a database of reactions, pathways and biological processes. Nucleic Acids Res. 39(1):D691-D697, 2011.
- C. Piller, Blots on a Field, Science 377(6604):358-363, 2022.