Stretch a rubber band far enough and it will break. Global healthcare systems are no different, and the forces stretching them are intense and increasing.
One emerging bright spot promises to ease the pressure, however: traditional and Generative AI (artificial intelligence) solutions that save time, reduce costs, relieve the burden on medical professionals, and – most promising and immediate – have the power to find new medicines and treatments in record time, saving lives at reduced costs.
What is stretching healthcare systems?
Why is healthcare experiencing such intense stresses? One big reason is that the world’s population is rapidly aging. The World Health Organization (WHO) reports that, in just 35 years, the percentage of people age 60 or older will nearly double, from 12% in 2015 to 22% in 2050; that’s 2.1 billion of the world’s 8 billion people. Those 80 or older will triple, to 426 million. Most troubling, by 2050 two-thirds of those over the age of 60 will live in low- and middle-income countries, where healthcare resources are already inadequate.
An aging population requires more care and suffers from more complex, chronic and specialized illnesses. But the healthcare professions aren’t growing fast enough to keep pace. By 2030, WHO estimates a shortfall of 10 million health workers, mostly in the same low- and middle-income countries with the largest proportion of elderly citizens.
The healthcare worker shortage is a dangerous recipe for human error. WHO estimates that the risk of injury from a medical error is already more than three times as great as the risk of being injured on an aircraft. About half of those errors are caused by administrative issues, and skyrocketing medical data volumes are creating massive administrative challenges. Consider: just one hospital can produce, on average, 50 petabytes of data annually. That’s double the entire content of the US Library of Congress, the world’s largest library – and the US alone had more than 6,000 hospitals in 2022.
That tsunami of data is overwhelming the world’s healthcare systems, but it could be a gold mine. For lurking in all that data – including difficult-to-decipher sources like MRIs, CT scans, X-rays and handwritten notes – are the patterns and insights that, if deciphered, could yield life-saving treatments and cures. The challenge is: how to find them?
How AI can help healthcare
Artificial Intelligence (AI) has two different forms: general AI, usually referred to as just AI, and Generative AI. Both are expected to play important roles in healthcare.
The difference? AI is best at spotting patterns that humans may miss, and then acting on those patterns based on rules set by its human programmers. It’s great for data analytics, such as finding the patterns in healthcare data relayed by wearable devices; automating complex, multi-step tasks to help healthcare workers do more tasks in less time, such as automating hospital record-keeping; and enhancing medical security systems with automatic updates. It can even track regional and global healthcare data to spot emerging pandemics and identify new viruses.
Generative AI goes further, enabling sophisticated modeling with a creative goal. It doesn’t just follow instructions; Generative AI can combine patterns to create something that never existed before.
“Instead of relying on specific rules, Generative AI models learn from the data and generate new content by capturing underlying patterns and relationships within the data,” upgrad.com reports. In the publishing and entertainment worlds, for example, debate is raging around Generative AI’s ability to “learn” existing authors’ writing styles and actors’ features and delivery to create new works with a human-like tone and style. A similar but more positive activity in healthcare: customizing medical devices for patients, such as automatically customizing a prosthetic design to a specific person’s height, weight and movements.
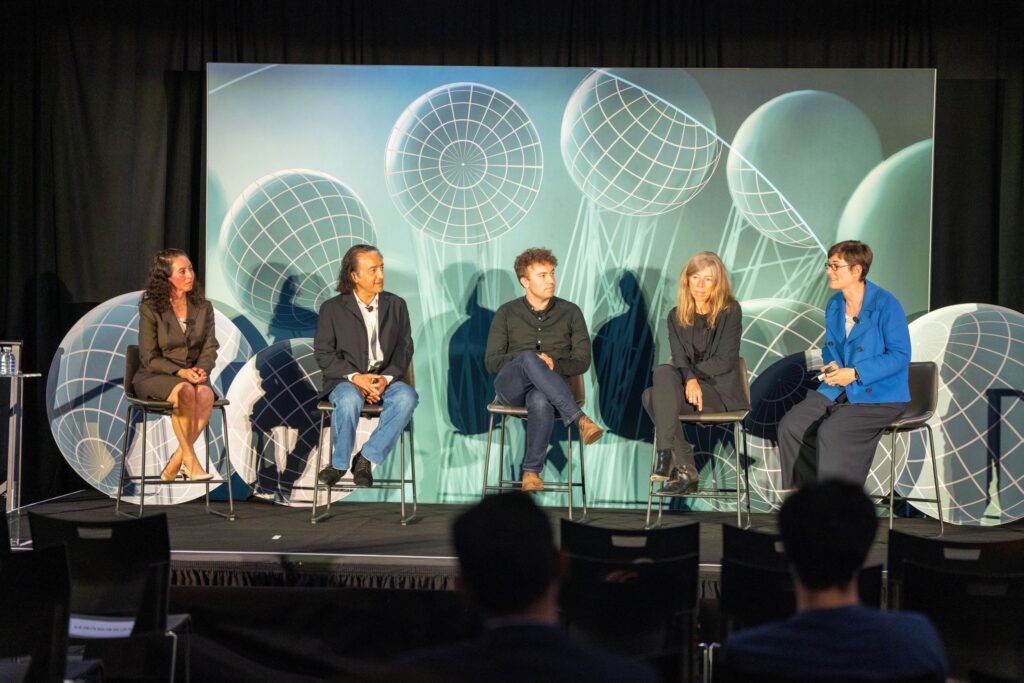
A few other healthcare applications for AI that the World Economic Forum identified in a May 2023 paper include:
- Assisting medical professionals with diagnoses. “Generative AI can analyze data from a patient’s medical records, lab results, previous treatments and medical imaging, such as MRIs and X-rays, to identify potential problem areas and suggest further testing and treatment options.
- Helping scientists study the effects of catastrophic health events, “such as modeling new pandemics and developing preventive measures.”
- Enabling predictive healthcare. Medical professionals don’t have time to wade through the data from all their patients’ wearable devices in search of emerging problems, but AI does. By predicting and managing crises before they occur, AI could help reduce the incidence of heart attacks, strokes and other crisis events.
Additional innovations identified by Boston Consulting Group (BCG) in a June 2023 paper include:
- Cancer detection. Paige.AI was the first company to receive FDA approval for AI use in digital pathology. It uses the technology to improve the efficiency and accuracy of prostate cancer diagnoses.
- Researchers have recently validated the use of digital modeling in clinical trials, including synthetic control groups.
- AI-powered manufacturing quality control software applications in hospitals and clinics can predict when equipment needs repair. Anticipating and scheduling maintenance before a failure would reduce downtime, relieve a common frustration for healthcare workers, and prevent disruption in daily operations.
Developing new drugs with Generative AI
Generative AI can go even further. Its ability to create new content from existing data is the key to one of the most exciting applications of AI to healthcare: inventing new medicines and finding new applications for existing medicines.
For example, BCG reports that Insilico Medicine used Generative AI to advance from target discovery to pre-clinical drug candidate for pulmonary fibrosis in just 18 months at a cost of only $2.6 million. Development of such drugs typically take a decade or more and can cost hundreds of millions.
“Human drug researchers tend to operate in a very limited design space, based on their experience with what works,” said Reza Sadeghi, chief strategy officer of BIOVIA, the scientific innovation brand of Dassault Systèmes. “But the available design space of drug like molecules is vast, it has been estimated that 1060 small molecules fit the criteria. AI has the advantage of fewer constraints or biases and through parallel processing virtually unlimited means to experiment. We’ve already seen examples of AI designing clinically successful drugs that no human would think to try.”
Generative AI also has found new applications for existing drugs, Sadeghi said, delivering new and better treatments for many illnesses with no investment in new research. “We’ve been doing this without AI, but the breakthroughs are much slower and fewer,” Sadeghi said. “AI creates efficiencies that translate into lower drug costs.”
Generative AI has the potential to multiply such benefits exponentially. Sadeghi outlines the problem: Half of all preliminary drug designs fail before they reach the detailed design stage, known as a lead; 90% of leads fail; and 90% of leads that reach the human clinical trial stage fail. Each failure costs pharmaceutical companies millions or even billions of dollars, contributing to the mind-numbing cost of those few that drugs that make it to market – especially those that will help relatively small groups.
For example, Sadeghi said, the first cure for sickle cell anemia is due to hit the market later this year. The cost: $2.5 million per patient. “Most of that cost came in the R&D phase,” Sadeghi said. “With Generative AI, we should be able to discover the next treatment more quickly and with less investment, which will make the treatment more affordable for more people.”
Specifically, Generative AI can help by accurately predicting the activity of the nearly 1 billion chemical compounds that can be combined to create medicines. By quickly identifying the compounds and combinations that will deliver the outcome a researcher wants, Generative AI can eliminate months or years of trial and error.
Before any research begins on a target compound, Generative AI also can predict whether that compound will have negative side effects, known in the industry as toxicity, Sadeghi said. If toxicity levels will be unacceptable, researchers can discard the compound as a candidate before investing any resources in R&D.
Protein folding with Generative AI
For example, the drug used for 30 years to treat Fabry, a genetic disease that causes a wide range of disorders, including chronic pain, fatigue, and problems with the heart, kidneys and gastrointestinal system, was extremely toxic. As an example of Generative AI’s promise, Lucas Nivon, CEO and co-founder of Cyrus Biotechnology, points to Fabrazyme, which used Generative AI to develop a better formulation.
Nivon calls himself “an AI optimist, but also an AI realist.” AI technology, he said at Dassault Systèmes’ 2023 Science in the Age of Experience forum, has important limitations that must be acknowledged and worked on. Still, he is willing to predict that, “in 10 years, most biologics will be partly or completely designed by algorithmic methods.”
To accelerate the transition, Nivon and his team are developing OpenFold, which uses Generative AI to understand the complex structures of the proteins that are the basis of all biology. Untangling these structures is a first step in developing successful pharmaceutical treatments.
While others have already released commercial protein-folding solutions based on Generative AI, they haven’t released the data and assumptions used to train the AI, Nivon said. This makes it difficult for researchers to apply it to projects using their in-house research data, because they know too little about the algorithms that drive its functions.
By revealing the entire training and inference stack behind its tool, OpenFold says its mission “is to bring the most powerful software ever created – AI systems with the ability to engineer the molecules of life – to everyone. These tools can be used by academics, biotech and pharmaceutical companies, or students learning to create the medicines of tomorrow.” In addition, OpenFold aims “to accelerate basic biological research and bring new cures to market that would be impossible to discover without AI.”
The work has such promising potential that Sadeghi contacted Nivon about using OpenFold to expand BIOVIA’s Generative Therapeutic Design module beyond chemistry-based small molecules. The alliance is a win-win arrangement, Sadeghi said: BIOVIA users gain access to a Generative AI tool that speeds up its pharmaceutical customers’ research, and OpenFold gains insights into the types of challenges that BIOVIA’s pharmaceutical customers are seeking to solve, helping to prioritize its work.
“OpenFold is already integrated into one of our drug design experiences, which we will be releasing to the marketplace near the end of 2023,” Sadeghi said. “We’re also working with the OpenFold organization to bring more capabilities to its platform to address the types of biologics that we believe our customers need.”
Resolving weak spots of AI in healthcare
For now, the errors that AI can make means that researchers must validate most of its findings with traditional physics-based methods to prove the AI has worked properly. Biased outputs, false results, and lack of insight into how AI arrives at its conclusions – a situation that non-proprietary approaches like OpenFold seek to combat – are common concerns today.
As the tools develop, however, and such issues are resolved, researchers will have to overcome their comfort levels with the tools they already know and move to the new methods, Sadeghi believes.
“When they see other companies bringing new treatments to market in two years instead of six, the economics will force them to change the way they work,” Sadeghi said. “The more success we have with AI, the more people will learn to trust it.”
The potential to accelerate the drug-discovery process is just too great to allow researchers to resist the change for long, he said.
“AI can reduce your process for identifying new candidate treatments from thousands of hours to 20 hours, and OpenFold can reduce that to two hours,” he said. “With pandemics, aging populations and underserved populations, that’s an improvement we must bring to fruition.”
Watch sessions from the Virtual Human Twin Experience Symposium now available on-demand.