The path to bringing a novel therapeutic to a patient is daunting with a lengthy and costly discovery process. Artificial Intelligence (AI) has revolutionized drug discovery, helping with these challenges, significantly reducing discovery timelines and associated R&D costs. More importantly, AI can enhance the quality of compounds progressing to clinical trials, increasing the success rates of new candidates. Recent breakthroughs in structural biology, chemistry, Machine Learning (ML), and the release of robust cloud computing platforms are turning the potential of AI in pharmaceutical industry into a reality.
Machine Learning Workbench – Democratize Target Modeling for Drug Discovery
1. What is Machine Learning Workbench?
Machine Learning Workbench is a cutting-edge, no-code application designed for data scientists and computational scientists working in the life sciences field. It offers a streamlined, intuitive solution for creating ML models and training pipelines on the 3DEXPERIENCE® platform.
Its key benefits include:
- QSAR and QSPR modeling using chemistry data
- Chemical feature calculation and selection
- Access to a wide range of powerful ML algorithms
- Hyper-parameter tuning for model optimization
- Model training and retraining with the possibility of training orchestration
- Easy data splitting and comprehensive model validation
- Models and training procedures version management
- Model publishing and sharing capability for democratization
2. Addressing Drug Discovery Challenges
Drug discovery requires precision and efficiency. Machine Learning Workbench improves team productivity by allowing researchers to create, manage and democratize QSAR models within their drug discovery teams through seamless integration to other 3DEXPERIENCE® platform applications. Medicinal/Computational chemists then leverage these models in BIOVIA Generative Therapeutics Design for improved lead optimization, predicting target/anti-target activity, toxicity or ADME properties with higher accuracy. Machine Learning Workbench also automates the training of models, thus enabling scalability, and frequent updates as new data becomes available.
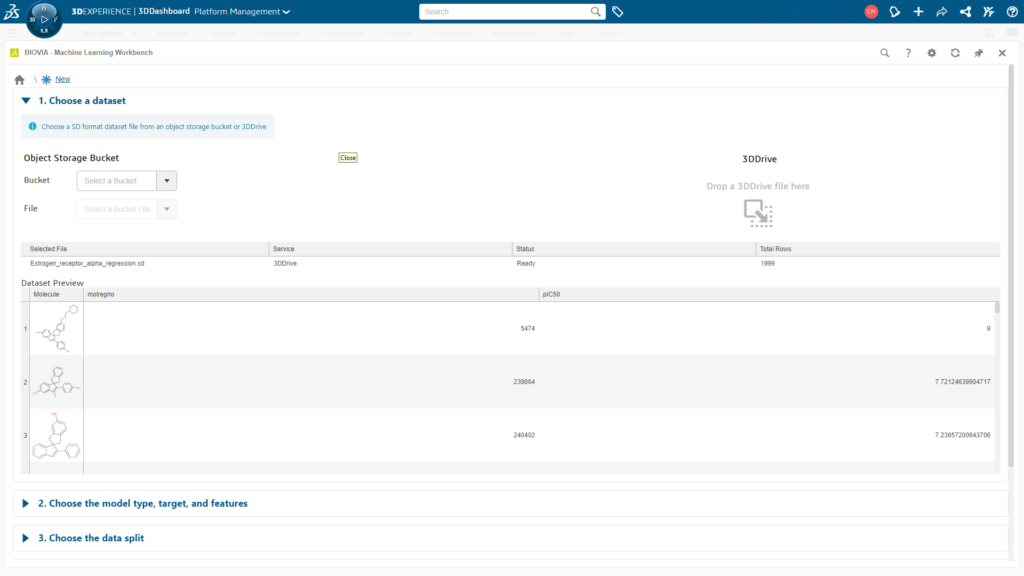
3. Example Use Case: Small Molecule Therapeutics Design for a Breast Cancer Target
Let’s explore a specific use case to illustrate the transformative potential of Machine Learning Workbench in drug discovery. In this example, we will focus on the development of new small molecule therapeutics for breast cancer that target the Estrogen alpha receptor.
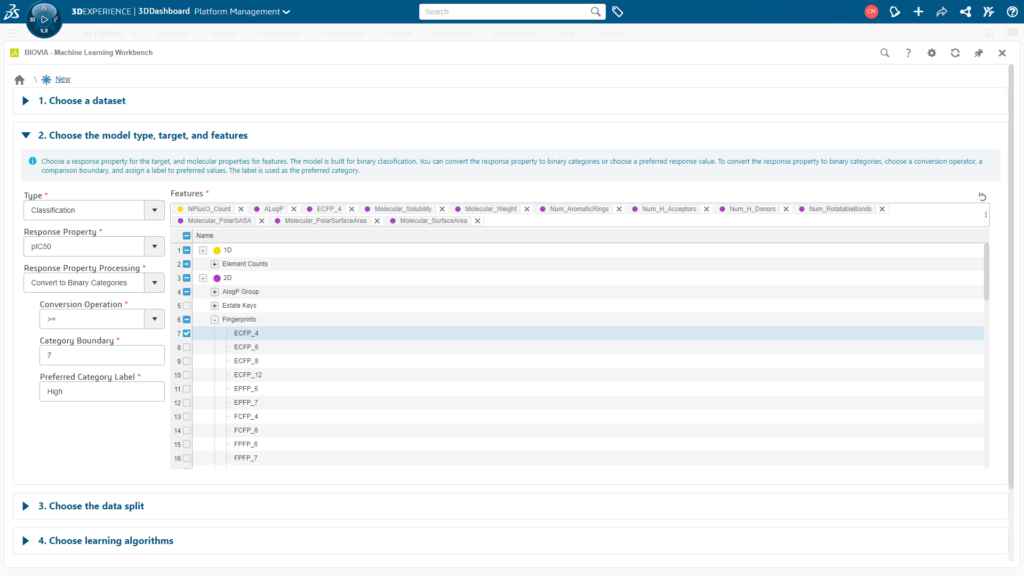
A data scientist starts with a dataset that includes various compounds, each detailed with its chemical structure and IC50 measurements. Utilizing Machine Learning Workbench, the data scientist creates a suite of diverse models with input features such as element counts, molecular weight, fingerprints, and many more. Each model employs different learners and hyper-parameters, aiming to predict the efficacy of these compounds against the target receptor (Figure 2). The data scientist then compares these models, eventually selecting, publishing and sharing the one that demonstrates the best performance. As soon as the model is shared with a computational chemist, he can screen potential drug candidates virtually.
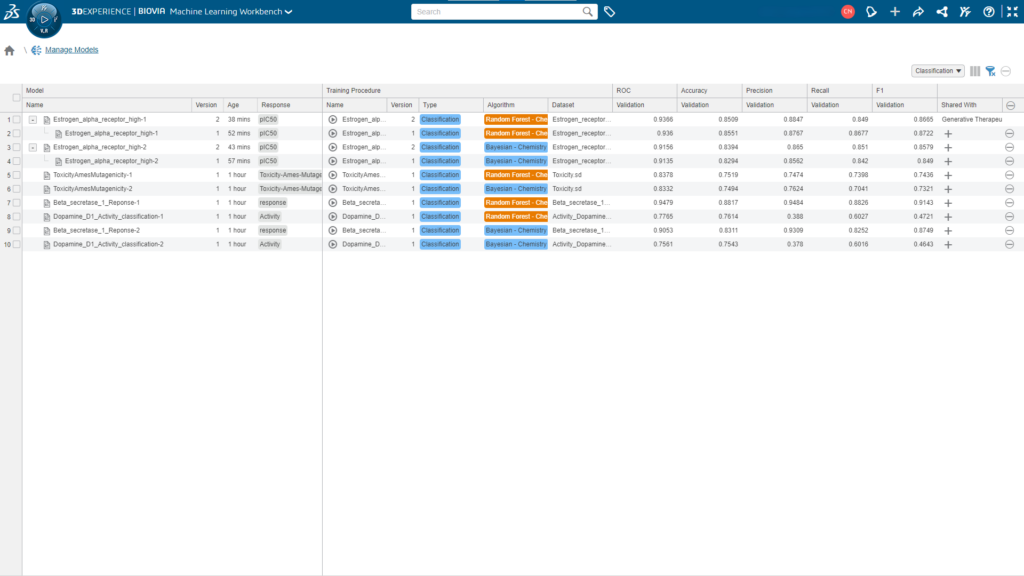
As the dataset is updated based on the new experimental IC50 measurements, the chosen model undergoes retraining, enhancing the model’s predictive accuracy. Now, the computational chemist can virtually screen potential drug candidates with a more accurate model. This iterative process, facilitated by Machine Learning Workbench, significantly reduces the need for physical testing, thereby accelerating the discovery and development of a small molecule inhibitor binding to the Estrogen alpha receptor.
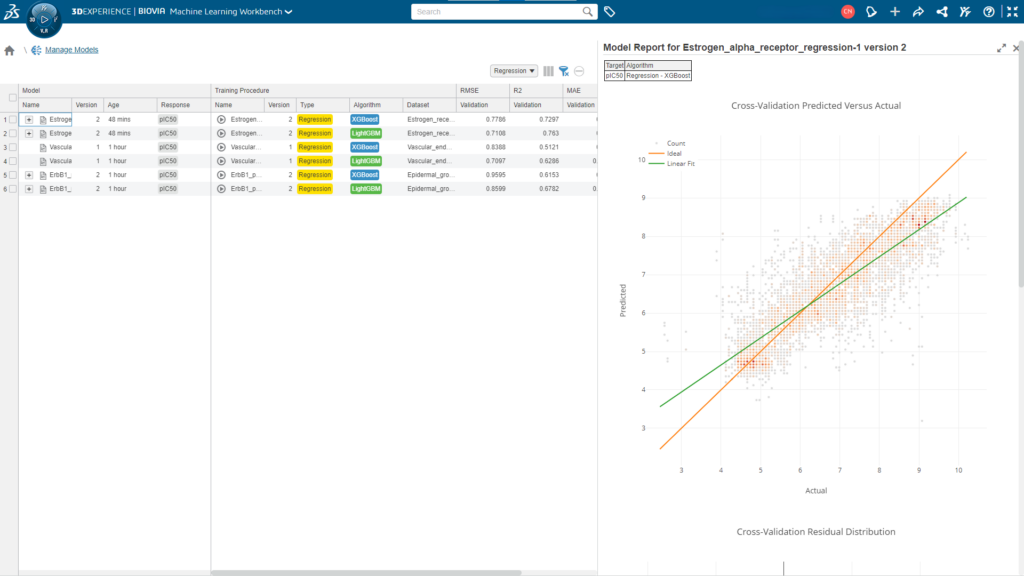
4. Democratizing Data Science in Drug Discovery
One of the most significant advantages of Machine Learning Workbench is its ease of use and democratization of ML models capability. It allows scientists with domain expertise and no programming skills to engage in sophisticated data science activities. They can build and share ML models with colleagues across teams and departments, fostering collaboration and collective innovation.
The Machine Learning Workbench is more than just a tool; it acts as a catalyst for change in drug discovery. By harnessing the power of AI and ML, it unlocks new horizons for researchers, empowering them to develop safer and more efficacious drugs more quickly and at lower costs compared to traditional methods. This marks the beginning of a new era in therapeutic development, an era where technology and human ingenuity unite to save lives.
Machine Learning Workbench is available through the Therapeutics Data Scientist Role in the 3DEXPERIENCE Platform. Watch the video for Therapeutics Data Scientist to discover how Machine Learning Workbench can transform your research.