Introduction
Lipid nanoparticles and membranes are pivotal in the advancement of drug delivery and biomedical engineering. Lipids, which form the structural basis of cell membranes, are involved in critical cellular processes such as endocytosis, mitosis, and membrane transport. The study of lipid membrane dynamics and their interactions with proteins and RNA is essential for developing innovative drug delivery systems. These nanoscale structures are essential in developing novel medicines due to their ability to encapsulate and efficiently deliver therapeutic agents1.
BIOVIA Materials Studio (MS) provides a comprehensive suite of tools for simulating and modeling complex systems, making it an invaluable resource in this field. By utilizing molecular dynamics methods, researchers can predict the behavior and performance of lipid-based systems in various biological environments. Coarse-grained (CG) simulations, in particular, offer a powerful approach to studying large systems efficiently, delivering accurate results that are otherwise difficult to achieve both with all-atom simulations and laboratory experiments, which are extremely time-consuming and costly.
In this context, BIOVIA Materials Studio software offers a way to use the Martini force field, which is well-known for its ability to simulate lipids. The latest version, Martini 3, brings significant advancements in lipid simulation parameters2. Integrating Martini 3 into MS allows for precise and efficient modeling of lipid bilayers, liposomes, and lipid nanoparticles.
In this study, reference studies from the literature were used to recreate simulations to verify the formation of bilayers and evaluate the effectiveness of Martini 3 within MS. Observing the self-assembly of 1,2-Dipalmitoylphosphatidylcholine (DPPC) into bilayers3, which naturally occurs at physiological temperatures, serves as a benchmark for assessing simulation accuracy.
Building on this foundation, the second phase of our study explores more complex systems. Utilizing MS, we investigate the formation and stability of liposomes considering the aspiration of their usage as carriers for vaccines, such as the Hepatitis B vaccine, which utilizes 1,2-Dioleoyl-sn-glycero-3-phosphoethanolamine (DOPE) and 1,2-Dioleoyl-sn-glycero-3-phosphocholine (DOPC)4,5. By constructing the coarse-grain model of these liposomes and analyzing them within water solution, we aim to demonstrate the competency and efficiency of MS and Martini 3 for modeling large liposomes.
Our overarching goal in this study is to leverage the capabilities of BIOVIA Materials Studio and the Martini 3 force field to optimize the formulation of lipid nanoparticles. This will ensure better control over their physicochemical properties, thereby enhancing their therapeutic achievement and contributing to the development of more effective drug delivery systems.
The simulations described in this blog were all ran on the 3DEXPERIENCE cloud using the Materials Studio Simulation application.
Using Materials Studio to Create Large Lipidic Systems
BIOVIA Materials Studio is particularly useful for constructing large and complex lipid systems due to its advanced simulation capabilities and user-friendly interface. It can handle very large systems, allowing researchers to study macroscopic properties and behaviors that are difficult to examine with smaller models. The integration of the Martini 3 force field enhances the accuracy of lipid simulations, providing reliable results that mimic real-world behaviors. Coarse-grained simulations in MS are computationally efficient, making it possible to perform large-scale studies in a reasonable time. Using the coarse graining tool from MS, we can transform an all-atom molecule to a coarse-grained one (figure 1). At the same time a force field file for the bead system, using parameters from the Martini 3 forcefield, can be generated.
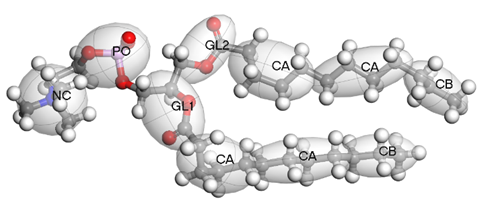
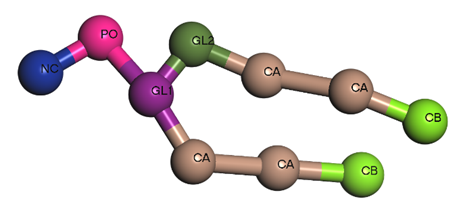
Figure 1 – DLPC molecule from all-atoms to coarse-grained model
After obtaining our coarse-grained molecule and setting its forcefield, we can generate mesoscale structures (Mesostructure) of various shapes. Materials Studio allows a user to create structures using the Mesostructure builder tool (figure 2). The user needs to enter the amounts of each coarse-grained molecules to go into each part of the structure.
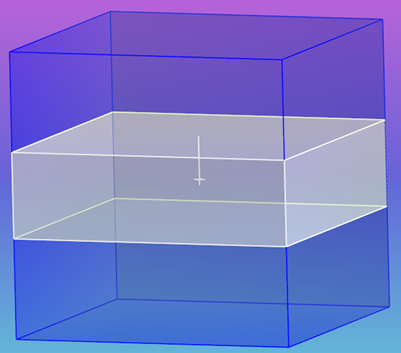
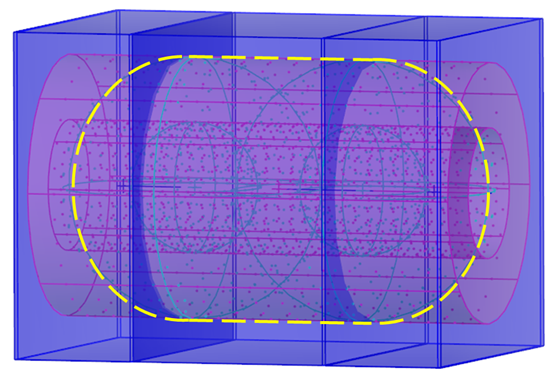
Figure 2 – Mesostructure builder
Using this tool, we can generate either a random mixture of molecules, like in figure 3, a homogeneous box filled with DPPC and Water.
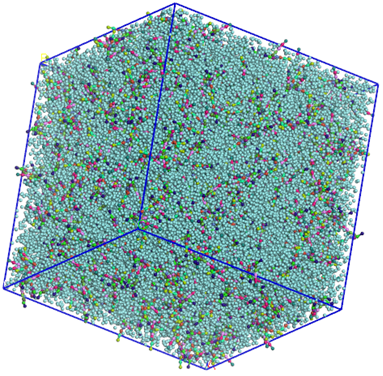
Figure 3 – Random mixture of DPPC and CG Water
Alternatively, molecules can be added to a predefined shape, as shown in figure 4 where we prebuilt a liposome made out of DOPE and DOPC (top), and a membrane made of DPPC (bottom).
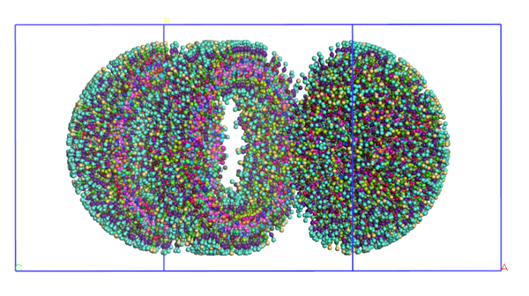
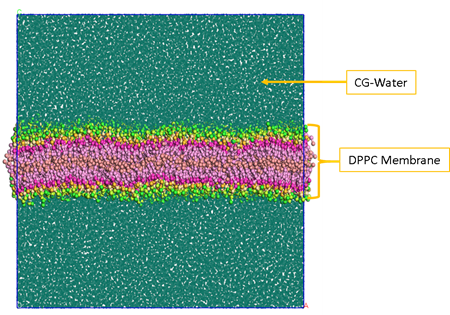
Figure 4 – Prebuilt liposome (top) and a prebuilt membrane (bottom)
As an example, the basic steps to create a system with a membrane are: create the box, add the slab shape, and define the type of molecule for each compartment of the box with their relative concentrations in the Mesostructure builder tool.
The video 1 below shows these steps while going a bit further for the creation of the liposome model step-by-step.
System Equilibration and Production Phase
Once we have created the models, we can start running the dynamics. Several steps are necessary before the main production phase:
- First geometry optimization with motion groups fixed,
- Second geometry optimization without the motion groups fixed,
- Short dynamic run (1000 ps), under NVT conditions with a time step of 0.1fs
- Main production run with a time step of up to 30 fs gradually increased from initial time step with consecutive NPT dynamics simulations.
The systems and conditions used during our work are presented in Table 1
System | Composition | FF | Run time (ns) | Box size (A) | Pressure (bar) | Temperature (K) | Time Step (fs) | Building |
I | 760 DPPC 70440 Water | Martini 3 | 300 | 350 x 212 x 128 | 1 | 325 | 30 | Pre-built |
II | 1600 DPPC 60000 Water | Martini 3 | 400 | 210 | 1 | 325 | 30 | Self-assembly |
III | 900 DOPE 2400 DOPC 305500 Water | Martini 3 | 1000 | 350 | 1 | 400 | 30 | Pre-built |
IV | 750 DOPE 2400 DOPC 180000 Water | Martini 3 | 250 | 300 | 1 | 400 | 30 | Self-assembly |
With the pre-built systems that corresponds to the membrane (I in the Table 1) and the liposome (III in the Table 1), we aim first to test the stability of the structures before letting them self-assemble from a random mixture.
Resulting Structures
After the main production phase, we check the stability of our structures by observing whether they maintain their membrane or liposome shapes throughout the run and whether the total energy of the system is equilibrated and remains stable during the run For the membrane, the membrane thickness was compared to the reference articles3,4. From the random mixture of DPPC and water (Figure 5), we obtained a membrane formation after 300 ns of run, consistent with the reference and used the Mesocite analysis tool to calculate concentration profile and measure the membrane thickness (Figure 5). Although the estimated thickness of the self-assembled membrane is slightly larger than the reference value at the considered paper, overall, the DPPC arranged themselves in a bilayer that remains stable along the simulation in agreement with the reference article.
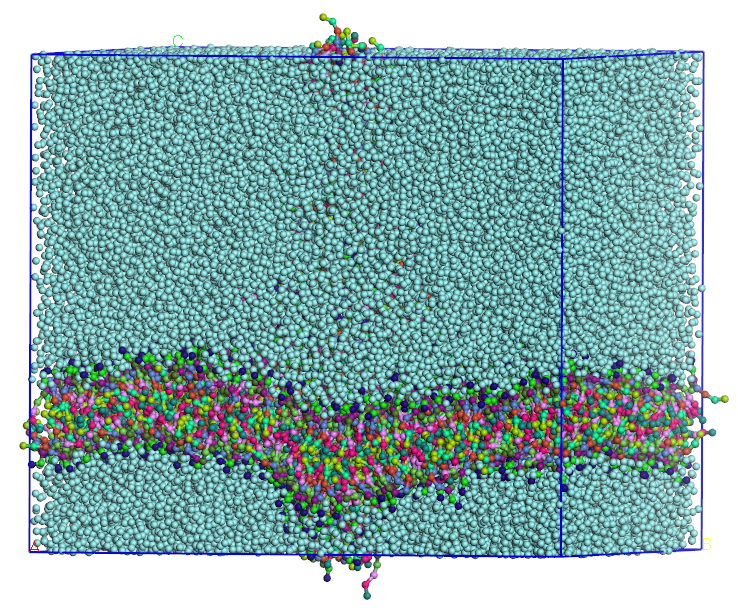
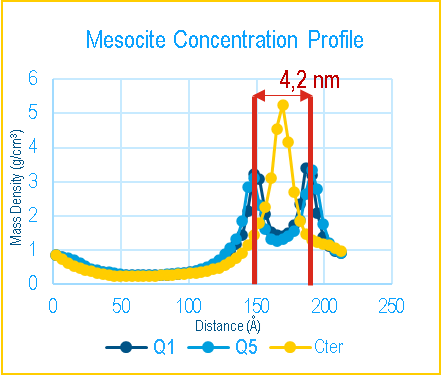
Figure 5 – Self formed membrane from DPPC (left) and Mesocite Concentration Profile on the DPPC Bilayer with the different head beads (Q1 and Q5) and tail beads (Cter) (right)
In Figure 6, you can see the resulting liposome from the prebuilt model after a 1000 ns dynamics simulation.
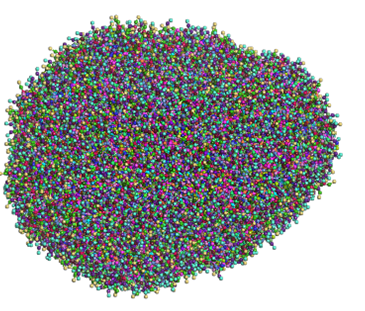
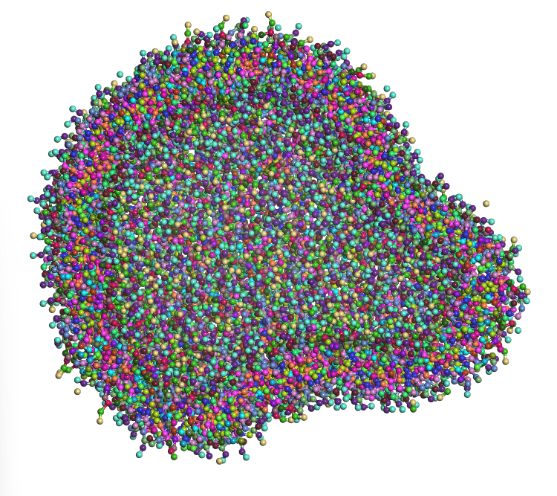
Figure 6 – Prebuilt liposome whole (top) and half cut (bottom) after a 1000 ns run (water was removed for better visibility)
In our simulations, the prebuilt models remained stable along the simulations, which encouraged us to run a random mixture of the lipids for each system and see if we could obtain the same structure during a self-formation.
From the random mixture of DOPE, DOPC and water, the liposome was almost completely formed after 1000 ns (Figure 6) of run, which was faster than the reference article.
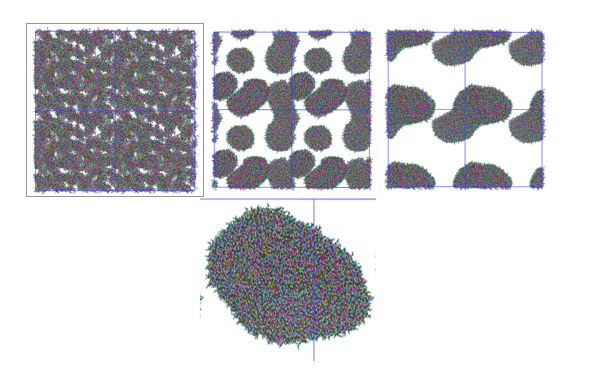
Figure 7 – Time laps of the liposome formation (t=1ns-165ns-400ns-800ns)
In Figure 7, you can see snapshots from the dynamics simulations of the liposome self-formation. Starting from a random lipid mixture, first lipids aggregate into small liposomes and then fuse into a single liposome.
The video below shows the formation of the liposome along the run.
Conclusion
The journey through simulating lipid nanoparticles and membranes using BIOVIA Materials Studio (MS) and the Martini 3 force field has been an exciting one. These tools open up new possibilities for understanding how lipid membranes function and how they can interact with proteins and RNA, which is key for developing better drug delivery systems.
Our experiments have shown that MS and Martini 3 are powerful allies in modeling lipid bilayers, liposomes, and nanoparticles. The ability to handle complex, large-scale simulations efficiently and fast has been a game changer. Observing and analyzing how our lipid membranes and liposomes come together in the simulations was particularly satisfying, confirming the accuracy of our methods.
Looking ahead, we are excited to expand our library of lipids for liposome formulation. By incorporating a wider variety of lipids, we aim to create more complex lipid nanoparticle recipes, enhancing their potential for effective drug delivery.
In short, BIOVIA Materials Studio and Martini 3 are great tools for anyone looking to dive into the world of lipid-based drug delivery. As we continue to explore and refine these techniques, we are optimistic about the future of creating more effective and targeted therapies.
Bibliography:
- Hou, X., Zaks, T., Langer, R., & Dong, Y. (2021). Lipid nanoparticles for mRNA delivery. Nature Reviews Materials, 6(12), 1078-1094.
- Souza, P. C., Alessandri, R., Barnoud, J., Thallmair, S., Faustino, I., Grünewald, F., … & Marrink, S. J. (2021). Martini 3: a general purpose force field for coarse-grained molecular dynamics. Nature methods, 18(4), 382-388.
- Marrink, S. J., De Vries, A. H., & Mark, A. E. (2004). Coarse grained model for semiquantitative lipid simulations. The Journal of Physical Chemistry B, 108(2), 750-760.
- Parchekani, J., Allahverdi, A., Taghdir, M., & Naderi-Manesh, H. (2022). Design and simulation of the liposomal model by using a coarse-grained molecular dynamics approach towards drug delivery goals. Scientific Reports, 12(1), 2371.
- Bulbake, U., Doppalapudi, S., Kommineni, N., & Khan, W. (2017). Liposomal formulations in clinical use: an updated review. Pharmaceutics, 9(2), 12.