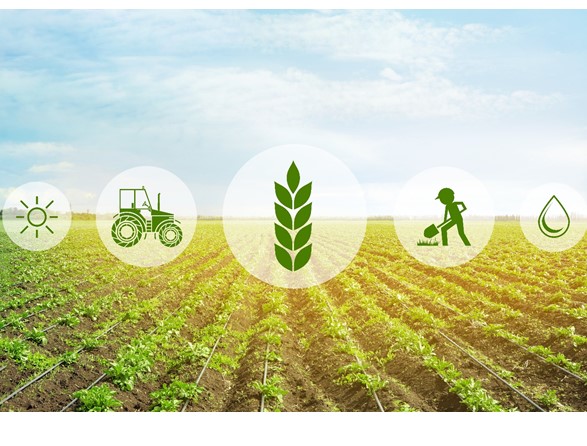
With the steady rise in population, the demand for food and fiber is predicted to grow rapidly. To keep up with the growing demand in the wake of diminishing arable land, exacerbated further by a changing climate, providing sustainable solutions in the agriculture sector behooves novel approaches. A successful second green revolution will entail technologies such as Artificial Intelligence (AI), Machine learning (ML) and Internet of Things (IoTs).
Digital agriculture initiatives have been put forward by several organizations with data collected from sensors, drones, and geographic information systems (GIS) of soil conditions, weather, and other factors that affect crop growth. Precision agriculture is a farming strategy that gathers real-time data, processes, and analyzes temporal, spatial and individual data and combines it with technology to optimize crop productivity. This enables precise decisions to improve resource use efficiency, productivity, quality, profitability, and sustainability of agricultural production. Precision agriculture, or precision farming, is a broad concept covering diverse IoT-driven approaches enabling farmers to manage farms and livestock with more control and accuracy. Precision agriculture is based on the idea that soil and plants are treated with extreme precision, and accurate farming methods are determined with the aid of smart farming technologies.
Data Science related technologies are revolutionizing several fields including Agriculture. IoTs play a major role in our daily lives, extending our perceptions and ability to modify the environment around us. Wireless sensors in fields are being used to collect data to monitor and control factors that influence crop growth and yield (Pang et al., 2015; Ojha et al., 2015; Talavera et al., 2017) and determine the optimum time to grow, harvest, detect diseases etc. (Ndzi et al., 2014).
Many startups and larger corporations are exploring various applications of IoTs in agriculture. Dassault Systèmes’ with 12 industry brands has a diversified portfolio for the digital transformation of agriculture. The BIOVIA brand within Dassault Systèmes supports the product portfolio as life sciences and molecular research & technology enabler, and thereby can cater to the needs of precision agriculture. The applications in BIOVIA are designed with a data science driven approach with a scalable framework to create, validate, and consume data science workflows. Democratizing data science helps teams to access and aggregate data from different sources to create simple to sophisticated analytics, and visualize with state-of-the-art reporting tools.
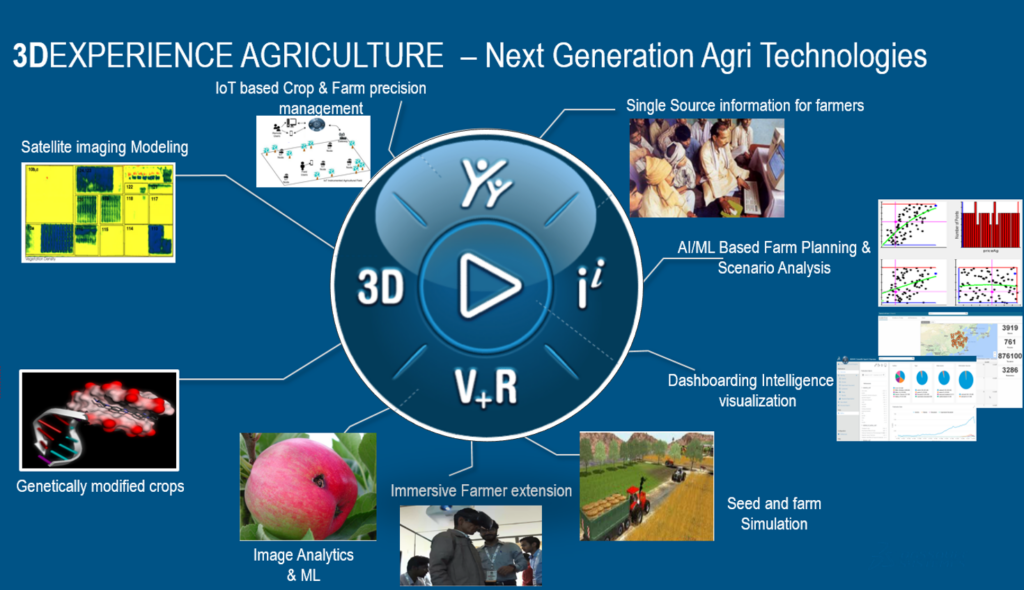
BIOVIA Pipeline Pilot application offers advanced data science, Machine learning (ML), AI in a democratized manner. Using Pipeline Pilot, complex datasets can be cleaned, explored for patterns, and machine-learning models can be generated easily with a few clicks. The ML model can be saved, shared and ultimately used for prediction. For precision agriculture, machine learning models can be built with data collected from soil, climate, crop types etc. This process can help farmers to decide what crops to sow, when to grow, how to protect from pests, weeds and others, when and where to market the produce to reap the maximum monetary benefits.
Here the ML model has been generated with a few Pipeline Pilot components with data comprised of soil, climate and rainfall (Dataset from Kaggle-Precision agriculture). This data can be used to build a predictive model to recommend the most suitable crops to grow in a particular farm based on the parameters shown below.
- N – Nitrogen content in soil
- P – Phosphorous content in soil
- K – Potassium content in soil
- Temperature – in degree Celsius
- Humidity – relative humidity in %
- pH – soil pH
- Rainfall- rainfall in mm
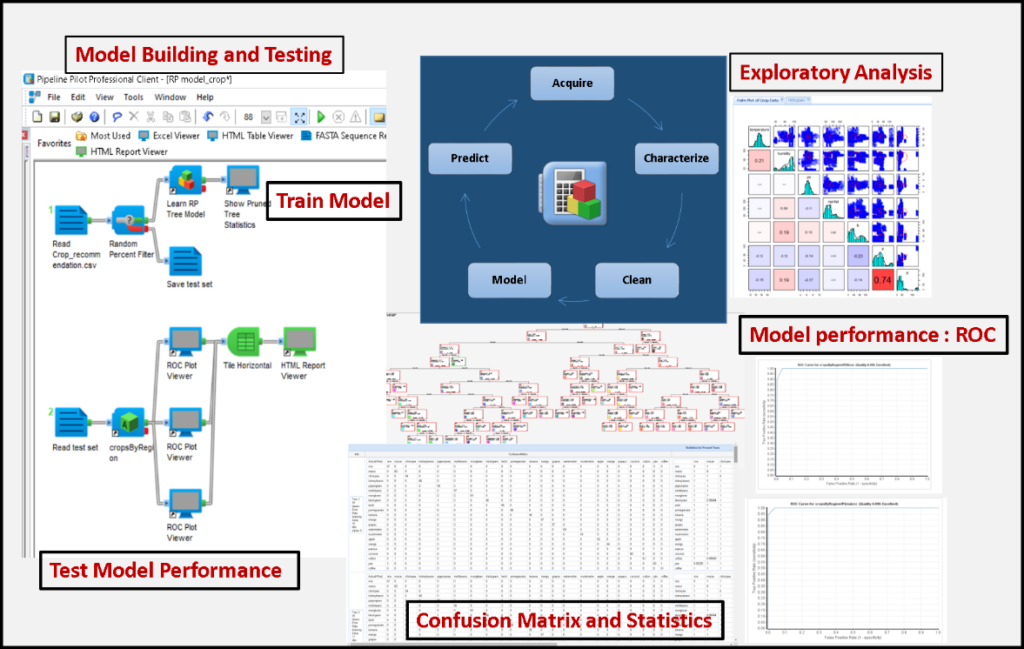
Pipeline Pilot offers machine learning and deep learning algorithms for classification, regression, and clustering analysis. Algorithms for several types of supervised, unsupervised and reinforcement learning algorithms are provided as Pipeline Pilot components. Expert users can also embed custom scripts from Python, Perl or R to maximize use across their organization.
For Precision Agriculture to become a reality, multiple technologies and algorithms must be available on a single platform that can integrate data from research labs and fields. To enable high productivity and reduced cost a real time data driven approach can be obtained by Dassault Systèmes 3D experience platform. Check our 3D experience platform that connects people, ideas, data, and solutions in collaborative environment for advancing sustainable agriculture production to feed the world.
About the Author:
Niranjani Iyer, PhD is a Data Scientist and Computational Biologist in BIOVIA, Dassault Systèmes. She has served in Industry and academics and has publications and patents focusing on different areas of Agriculture research.
References
Pang, Z., Chen, Q., Han, W., Zheng, L., 2015. Value-centric design of the internet-of things solution for food supply Chain: value creation, sensor portfolio and information fusion. Inform. Syst. Front. 17, 289–319.
Ndzi, D.L., Harun, A., Ramli, F.M., Kamarudin, M.L., Zakaria, A., Shakaff, A.Y.M., Farook, R.S.,2014. Wireless sensor network coverage measurement and planning in mixed crop farming. Comput. Electron. Agric. 105, 83–94.
Ojha, T., Misra, S., Raghuwanshi, N.S., 2015. Wireless sensor networks for agriculture: the state of-the-art in practice and future challenges. Comput. Electron. Agric. 118, 66–84.
Talavera, J.M., Tobón, L.E., Gómez, J.A., Culman, M.A., Aranda, J.M., Parra, D.T., Garreta, L.E., 2017. Review of IoT applications in agro-industrial and environmental fields. Comput. Electron. Agric. 142, 283–297