The question of what should we make next has challenged the world of drug discovery for decades. For years, computational methods for small molecule drug design have offered numerous algorithms and methodologies to help generate new ideas and guide the iterative process of lead design and optimization. For a particular drug target, these methods help to identify high-quality candidates that may eventually advance to clinical development with less experiments and time in the lab. From the early days of combinatorial chemistry and bioisosteric replacement to ligand-, fragment- and structure-based design, there have been many tools, leveraging numerous algorithms that suit your project constraints and design criteria. More recently, AI and machine learning algorithms have been popular in allowing researchers to rapidly explore more ideas in the chemical space and propose novel structures that a medicinal chemist may not have considered trying out when looking for new drugs.
Until recently, the computational design tools for biotherapeutics seemed to require more expertise, and to be more sparse and application-specific compared to the tools that exist for small molecule therapeutics. Of course, there are computational design algorithms available such as homology modeling, protein-protein docking and combinatorial scanning mutagenesis for general protein modeling and binder design, which are used in biotherapeutics lead discovery and optimization. For designing certain types of biological therapies, such as monoclonal antibodies, there are methods such as affinity maturation, humanization and immunogenicity prediction algorithms. However, to help answer directly what variation of our biotherapeutic we should make and test next, two recent AI methods, RFDiffusion and ProteinMPNN, have totally changed the nature of biotherapeutics discovery. These tools have the potential change the way we design biotherapeutics by helping to identify novel candidates that the computational and molecular biologists may not have considered.
Generating Proteins with AI: RFDiffusion and ProteinMPNN
RFDiffusion is a cutting-edge generative AI algorithm that can “diffuse” a collection of amino acids into a protein structure. The diffusion process starts with a random, noisy collection of atoms and, through a series of controlled refinements the algorithm makes adjustments to the structure to reduce the noise and move closer to a biologically realistic and functional protein structure. One common analogy for the diffusion process is developing a photo from a blurry image; iterative processing steps can take an initial grainy image and refine the detail and clarity to produce a final clear picture.
RFDiffusion can be utilized for a number of different biotherapeutic design challenges, such as engineering a biologic that can bind to a viral protein to neutralize the virus. With antibody structures or other protein-protein systems, RFDiffusion can be used to design new protein scaffolds that may improve binding affinities or enhance the stability of the binding partners. RFDiffusion can be also used to generate enzyme therapeutics that may break down a specific substrate to treat metabolic disorders. Beyond biotherapeutics, RFDiffusion has potential to help design proteins for industrial and biotechnological applications such as making enzymes that catalyze specific chemical reactions or proteins that suit very specific conditions including low or high temperature, pH, etc.
ProteinMPNN is a state-of-the-art neural network that can predict one or more probable protein sequences given a protein structure. This algorithm has been published with success in one of the most critical aspects of protein sequence design – generating sequences that fold into a stable protein/peptide with propensity to crystallize, facilitating the structure determination of these proteins. ProteinMPNN can be used in conjunction with RFDiffusion to generate new protein designs such as new enzymes or antibodies that can be further evaluated for desired properties such as stability, activity, affinity, and specificity. One of the strengths of ProteinMPNN is its ability to generate multiple sequence variants. This ability is invaluable as different variants provide more options to test and identify candidates with the best performance in terms of efficacy, safety, and manufacturability. Just as significantly, these variants also provide alternative leads when candidates encounter unforeseen issues in protein optimization, during protein expression, or ADMET challenges such as solubility and immunogenicity.
Together, RFDiffusion and ProteinMPNN significantly expand the biological space that can be explored in silico before biologists need to commit to expensive and time-consuming physical experimentation. They have the potential to open up exciting avenues for more intelligent, model- and data-driven workflows driving innovation in biotherapeutic design.
Generating Proteins with RFDiffusion and ProteinMPNN in Discovery Studio Simulation
In BIOVIA Discovery Studio Simulation, a new Generate Protein Scaffolds protocol now provides easy access to RFDiffusion workflows, the first of which is motif scaffolding. Users can start with a specific part of an existing protein (the motif) and design a complete new protein scaffold that incorporates this motif. This approach allows precise control over the functional regions of the protein, as well as control over the protein scaffold design, via different model weights that suit particular proteins and complexes.
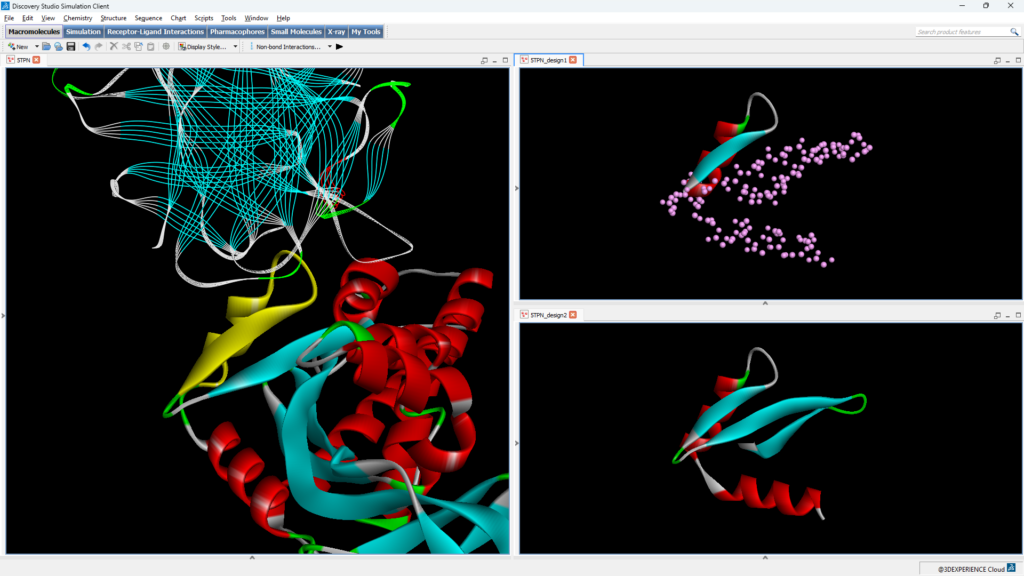
A second new protocol, Generate Protein Sequences, allows users access to not only ProteinMPNN, where they can easily define sequence residues for design, but also to LigandMPNN and SolubleMPNN models. LigandMPNN is an extension to ProteinMPNN that is able to consider protein, small-molecule, nucleic acid, and metal ion ligands as additional context for designing sequences, with the potential to improve the chemical properties of the designed sequences. SolubleMPNN could be a better model to use when protein solubility is part of your design criteria. Users can determine the degree of sequence diversity and confidence desired, as part of the generative design, and have the ability to control the bias of particular amino acids.
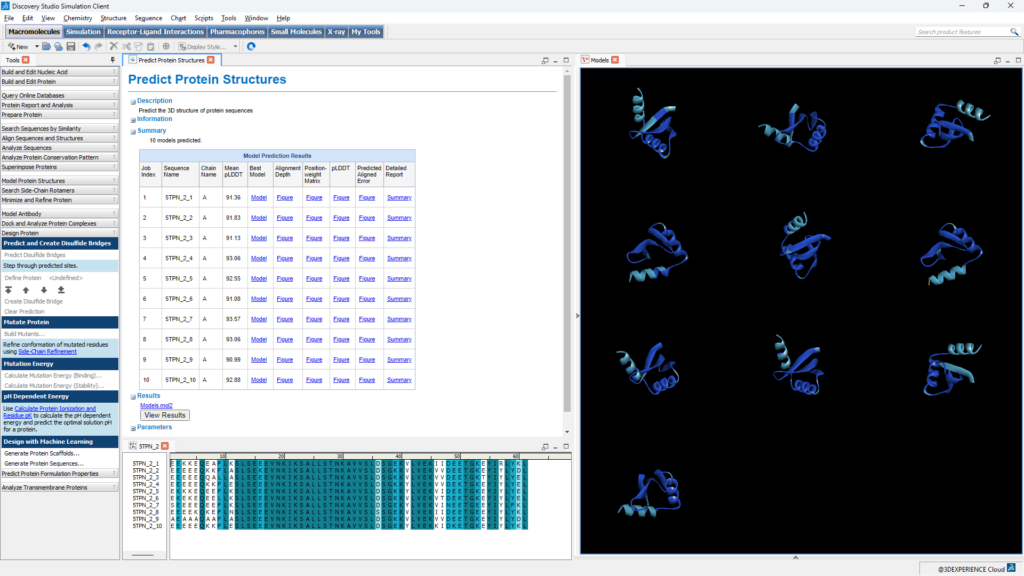
These two significant new enhancements are exciting additions to the biotherapeutics and protein design tools in Discovery Studio Simulation in the 3DEXPERIENCE® Cloud, which already includes AlphaFold and OpenFold AI structure prediction. They expand the ever-growing arsenal of powerful AI tools for molecular modelers and biologists to help answer the question of “what to make and test next” and accelerate the rational design of biologics. In combination with the existing physics-based methods in Discovery Studio Simulation, users can rapidly explore many more possibilities in silico before arriving at the final handful of candidates that are ready to become a successful commercial biotherapeutic or a biological to be used in agriculture, food and beverage, or environmental industries.
Nobel Prizes in Chemistry and Physics
This year’s Nobel Prizes in Chemistry and Physics celebrate how AI is pushing the boundaries of scientific research. John J. Hopfield and Geoffrey E. Hinton were awarded the Nobel Prize in Physics for their foundational discoveries in machine learning with artificial neural networks, while David Baker, Demis Hassabis, and John Jumper received the Nobel Prize in Chemistry for breakthroughs in computational protein design and protein structure prediction.
At BIOVIA, we are proud to be part of this AI revolution. By integrating AlphaFold2, OpenFold, RFDiffusion, and the ProteinMPNN family of models into our platform, we empower researchers with cutting-edge tools for protein structure prediction and protein design.
Watch the video to learn more how Discovery Studio Simulation now helps users generate novel biologics with RFDiffusion and LigandMPNN models.